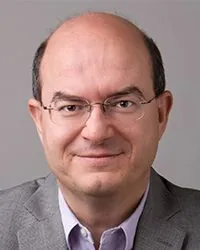
How AI Could Transform Identification of MASH Patients Eligible for Resmetirom
2024-12-15
Author: Jacob
Introduction
Artificial intelligence is making waves in the medical field, and new research suggests it could be a game changer for screening patients with metabolic dysfunction-associated steatohepatitis (MASH) who may qualify for resmetirom treatment. This groundbreaking study, presented at the 22nd Annual World Congress on Insulin Resistance, Diabetes & Cardiovascular Disease (WCIRDC), highlights the current inefficiencies in non-invasive screening methods used to detect noncirrhotic MASH patients with moderate-to-severe liver fibrosis.
Need for Better Methodologies
Lead researcher Dr. Christos Mantzoros, an endocrinologist at Beth Israel Deaconess Medical Center, pointed out the urgent need for better methodologies. Existing non-invasive tests have been found wanting, particularly when it comes to identifying MASH stages F2 to F3 without cirrhosis. The study’s findings advocate for the integration of machine learning models to enhance diagnostic accuracy, sensitivity, and predictive capabilities, offering a potential lifeline for numerous patients who are often overlooked due to inadequate testing.
Resurgence of Hope in Hepatology
After a decade-long dry spell without effective treatments, the hepatology community has experienced a resurgence of hope following the FDA's historic approval of resmetirom as the first therapy targeting noncirrhotic nonalcoholic steatohepatitis with moderate to advanced fibrosis. This monumental milestone led by Madrigal Pharmaceuticals came about after an extensive clinical development program involving 12 phase 1 studies, two phase 2 studies, and four phase 3 studies.
Enhancing Diagnostic Value
In their research, Mantzoros and colleagues utilized a comprehensive multinational biobank of 905 biopsy-confirmed participants, mirroring a diverse representation of MASH and healthy control groups. Through a rigorous analysis involving 28 different non-invasive tests—including biomarker assessments and imaging techniques—they aimed to enhance the diagnostic value of existing methodologies.
Impressive Results of Machine Learning Models
What’s particularly impressive is the development of clinical and hormonal-based machine learning models that achieved significant improvements over traditional testing. These models yielded an area under the curve (AUC) of 0.86, sensitivity of 93%, and a negative predictive value (NPV) of 97%, greatly surpassing prior non-invasive test benchmarks. The study further emphasizes the gains made when adding key variables like aminotransferases and components of metabolic syndrome, which elevated the AUC to 0.91.
Cirrhosis Detection Challenges
In addressing the challenge of cirrhosis detection, the study found that standard tests like AGILE4+ showed respectable results with sensitivity rates climbing to 92%. Yet, intriguing results from machine learning models soared even higher, exhibiting an AUC near 0.98 and sensitivity hitting 98%.
Conclusion
As we prepare to face the future of hepatology in 2025, the integration of sophisticated AI tools and novel screening techniques could streamline patient identification processes, ensuring that candidates for life-changing therapies like resmetirom are recognized and addressed more effectively than ever before.