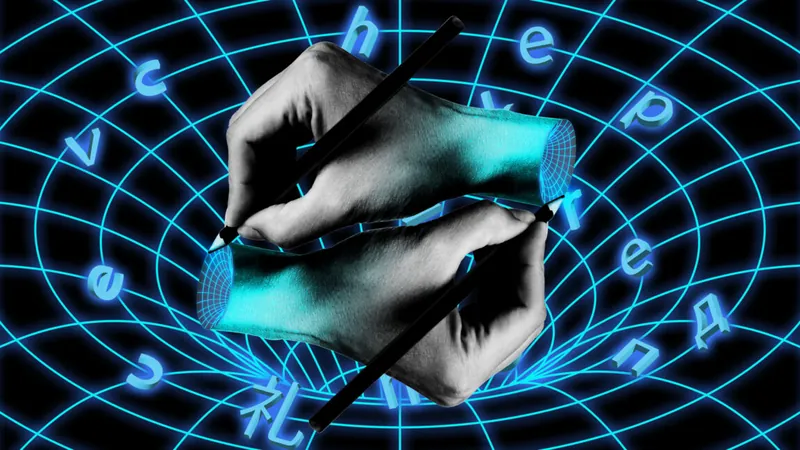
New Methods Empower Hackers to Launch Advanced Attacks on AI Models Like Google’s Gemini
2025-03-28
Author: Olivia
Introduction
In an alarming advancement for cybercriminals, researchers have revealed that indirect prompt injection attacks can be significantly enhanced, particularly against large language models (LLMs) such as Google’s Gemini and OpenAI’s GPT series. These attacks find vulnerabilities in the system by manipulating the model’s inability to distinguish between user prompts defined by developers and external text it interacts with. As a result, attackers leverage this weakness to provoke harmful actions, such as accessing confidential user data or delivering misleading responses that can disrupt crucial calculations.
Traditional Attacks vs. New Techniques
Traditionally, prompt injection attacks required extensive manual effort to craft effective prompts, especially against closed-weight models where the underlying code and data remain proprietary and closely guarded. This makes it difficult for attackers to devise successful methods due to the opaque nature of these models.
The Fun-Tuning Breakthrough
However, groundbreaking techniques by academic researchers reveal a novel approach that could change the game. They have created a method to generate optimized prompt injections for Gemini that boast notably higher success rates compared to manual methods. By exploiting a feature of these models known as fine-tuning—commonly used to tailor LLMs on specific datasets—hackers can effectively train their prompts to work more efficiently.
Methodology of Fun-Tuning
The researchers, who have coined their algorithmic approach as "Fun-Tuning," employ discrete optimization to enhance prompt injections systematically. This method allows attackers to conduct automated searches for effective configurations rather than relying solely on trial and error. As a result, crafting successful injections has transitioned from an art form into a more scientific endeavor that could yield quicker results.
Cost and Efficiency
For instance, Fun-Tuning can modify a simple prompt injection with additional prefixes and suffixes, enabling it to bypass safeguards that would have otherwise blocked the action. This process requires around 60 hours of compute time on Gemini’s free fine-tuning API, with an estimated cost of about $10 for successful execution, making it accessible for skilled attackers.
Success Rates and Implications
Results from the research indicate that optimized prompt injections generated through Fun-Tuning achieved impressive success rates of 65% against Gemini 1.5 Flash and 82% against Gemini 1.0 Pro, compared to baseline rates of only 28% and 43%. Furthermore, these successful attacks could easily transfer from one Gemini model to another, raising alarms about the robustness of protections across different iterations of the LLM.
Responses from Google
While Google has recognized the potential risks associated with prompt injection attacks, they maintain that ongoing efforts to bolster defenses are in place, including regular red-teaming exercises designed to expose vulnerabilities. Nonetheless, the more systemic nature of Fun-Tuning poses additional challenges for developers as it exploits fundamental elements of the fine-tuning process used to enhance model performance.
Broader Implications for AI Security
The implications of this research extend beyond just the Gemini models; it suggests a growing sophistication in cyber-attack strategies targeting artificial intelligence systems at large. The results of these studies will be presented in May at the IEEE Symposium on Security and Privacy, igniting conversations about the balance between model utility for developers and adequate security measures.
Conclusion
As the landscape of AI security evolves, understanding the strengths and weaknesses of AI systems like Gemini will be pivotal for protecting sensitive information and maintaining the integrity of AI-powered services. The revelations surrounding Fun-Tuning may serve as a wake-up call for stakeholders in technology and cybersecurity to rethink their strategies for defending against increasingly advanced attacks.