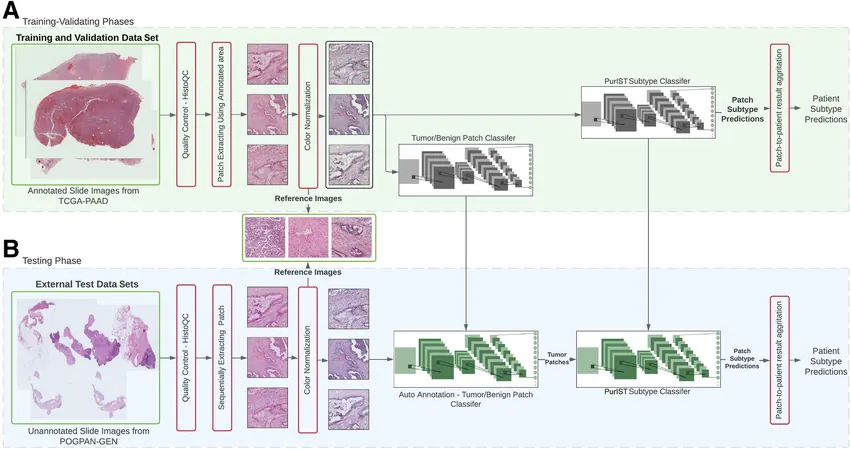
Revolutionary AI Tool Transforms Pancreatic Cancer Diagnostics: What You Need to Know!
2024-12-12
Author: Olivia
Breakthrough in Pancreatic Cancer Diagnostics
In a groundbreaking advancement for pancreatic cancer diagnostics, researchers have developed an innovative deep learning model that accurately classifies pancreatic ductal adenocarcinoma (PDAC)—the most prevalent type of pancreatic cancer—into distinct molecular subtypes using standard histopathology images. This remarkable technique not only offers a high degree of accuracy but also presents a speedy and cost-effective alternative to traditional methods reliant on pricey molecular assays.
Published in the esteemed American Journal of Pathology, this pivotal study holds the promise of refining personalized treatment strategies, potentially improving patient outcomes significantly.
Urgent Need for Early Detection
PDAC has recently surged past breast cancer as the third leading cause of cancer-related deaths in both Canada and the United States. Early detection can enable surgical intervention, curing about 20% of PDAC cases; however, the sobering reality is that the five-year survival rate for patients remains stuck at a mere 20%. Alarmingly, by the time of diagnosis, roughly 80% of patients already have metastatic disease, with most succumbing within a year.
Challenges in Current Methodologies
The aggressiveness of PDAC presents a daunting challenge when employing sequencing technologies to tailor patient care plans. Given the urgency implied by the disease’s rapid progression, immediate identification of patients suitable for targeted therapies and clinical trials is crucial. Unfortunately, existing molecular profiling processes take between 19 to 52 days post-biopsy, which fails to align with the critical time demands posed by the disease.
David Schaeffer, MD, co-lead investigator from the Department of Pathology and Laboratory Medicine at the University of British Columbia, highlights, 'As more actionable subtypes emerge for personalizing pancreatic cancer treatment, current subtyping relies heavily on genomic methodologies that analyze DNA and RNA from tissue samples.'
Introducing a New Solution
He elaborates, 'This approach is effective only when there’s enough tissue to work with—a significant obstacle in the case of PDAC due to the organ's challenging anatomical position. Our study introduces a promising method for rapidly and affordably classifying PDAC molecular subtypes from routine hematoxylin-eosin (H&E) stained slides, which could greatly enhance clinical management.'
To achieve this, the researchers trained AI models using whole-slide pathology images to identify PDAC molecular subtypes, specifically the basal-like and classical varieties. Notably, H&E staining is a widely practiced, low-cost technique regularly utilized in pathology labs for diagnostic and prognostic purposes.
Results and Accuracy
The developmental phase featured training these AI models on 97 slides from The Cancer Genome Atlas (TCGA) while testing their accuracy on 110 slides from 44 patients in a local cohort. Impressively, the most effective model reached a jaw-dropping accuracy of 96.19% for subtype identification in the TCGA data and 83.03% in the local patient group, underscoring the model's reliability across varied datasets.
Future Implications
Ali Bashashati, Ph.D., co-lead investigator from the School of Biomedical Engineering, underscores the model's utility, stating, 'The tool exhibits a sensitivity of 85% and a specificity of 100%, marking it as an exceptionally viable candidate for prioritizing patients for molecular testing. The primary highlight of our study is that this AI model can identify subtypes directly from biopsy images, making it a practical asset at the time of diagnosis.'
The implications of this research are immense, opening doors to faster and more efficient diagnostic methods that could save lives. With pancreatic cancer's notorious reputation and limited treatment options, this AI advancement could be the game-changer the medical community has been waiting for. Keep your eyes peeled—this could redefine the landscape of pancreatic cancer management!