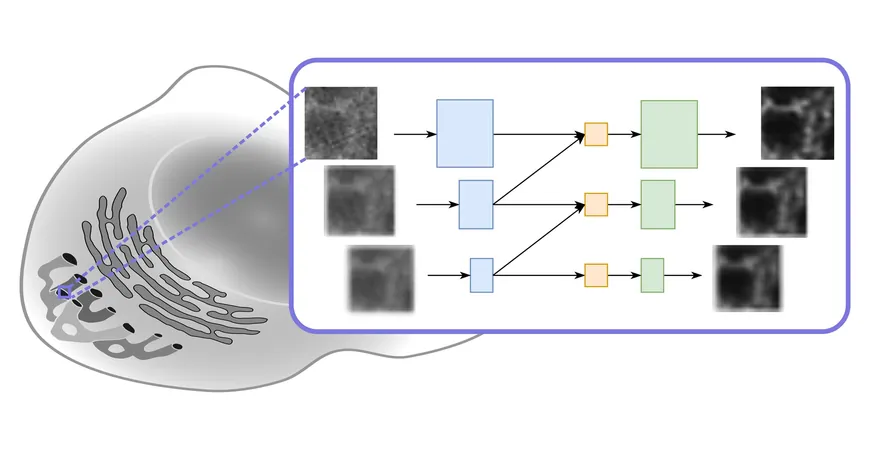
Revolutionary Deep Learning Architecture Transforms Light Microscopy Image Processing
2024-11-19
Author: Charlotte
Introduction
In the realm of light microscopy, the challenge of revealing intricate details has been tackled with increasing sophistication. However, the quest for enhanced image contrast and resolution remains an ongoing priority within the scientific community. Enter a groundbreaking advancement—a cutting-edge deep learning architecture developed by pioneering researchers at the Center for Advanced Systems Understanding (CASUS) and the Max Delbrück Center for Molecular Medicine.
The Multi-Stage Residual-BCR Net (m-rBCR)
Dubbed the Multi-Stage Residual-BCR Net (m-rBCR), this innovative model not only operates faster than its traditional counterparts, but also matches and often surpasses their image quality. This significant breakthrough was first unveiled at the prestigious European Conference on Computer Vision (ECCV) and is detailed in a forthcoming paper for the 2024 proceedings.
Deconvolution Technique
At the core of this advancement is a computational technique known as deconvolution, a process designed to enhance contrast and resolution in images captured by various types of optical microscopes—including widefield, confocal, and transmission microscopes. Deconvolution addresses the common issue of image blur, which arises from optical systems that capture light from out-of-focus structures.
Challenges with Conventional Techniques
Dr. Artur Yakimovich, the lead researcher and head of a CASUS Young Investigator Group, explains, “The principal challenge with conventional PSF (point spread function)-based deconvolution techniques lies in the difficulty of accurately obtaining the PSF of a given microscopic system.” This has led to significant research on blind deconvolution methods, where the PSF is inferred directly from the images instead of known a priori—a task that has proven to be fraught with complexity and limited progress.
Innovative Solution: Physics-Informed Neural Network
Consequently, the Yakimovich team has proposed a novel solution using a physics-informed neural network tailored for microscopy challenges. By employing the m-rBCR model, they effectively address the inverse problem associated with image reconstruction, significantly improving the final output's quality.
Key Features of m-rBCR Model
A distinctive feature of the m-rBCR model is its use of the frequency representation of images over the conventional spatial format, leveraging the model's ability to process monochromatic microscopy images more effectively. “By utilizing the frequency domain, we can achieve optically meaningful data representations, allowing for impressive deconvolution with surprisingly few parameters compared to other deep learning architectures,” Rui Li, the first author of the study, explains.
Validation and Performance
Success was validated through extensive testing on four diverse datasets—two simulated and two real microscopy datasets—showing that m-rBCR dramatically outperformed other contemporary deep learning models while requiring fewer resources.
Lightweight Design and Future Aspirations
What constitutes a pivotal benefit of this architecture is its lightweight design, which deviates from the trend of developing increasingly large models that demand overwhelming computational power. As Prof. Misha Kudryashev notes, “Our model adeptly reduces superfluous parameters without compromising performance, making it uniquely suited for microscopy applications.”
Looking ahead, the Yakimovich research group aims to further expand the capabilities of machine learning in the biomedical field. Their recent work on a Conditional Variational Diffusion Model has also set new benchmarks in image quality enhancement. However, the computational demand for such advanced models is substantial. In contrast, the m-rBCR model circumvents these requirements whilst still achieving high-quality results.
Conclusion
With aspirations to make the m-rBCR model user-friendly for the imaging community, this technological leap is set to redefine how scientists analyze and interpret microscopic imagery. As researchers continue to unlock the complexities of molecular interactions within biological systems, the integration of advanced machine learning techniques will be pivotal in propelling these studies forward, bringing unprecedented clarity and precision to the world of microscopy.
Stay tuned to witness how these innovations will reshape diagnostic processes, 3D image reconstruction, and ultimately enhance our understanding of disease mechanisms!