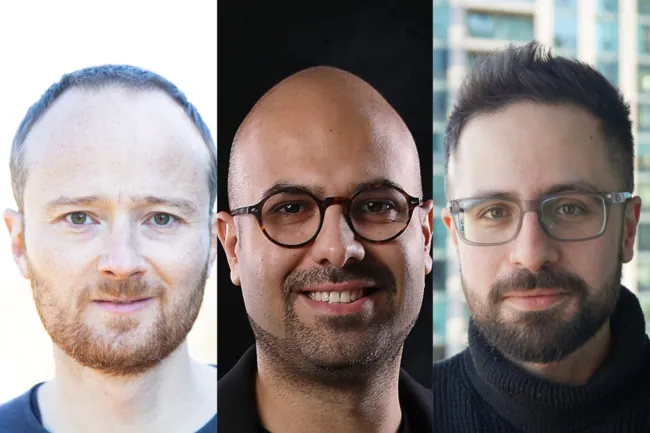
Revolutionary Machine Learning Method Reveals Hidden Patterns in Human Genome Organization – What This Means for Precision Medicine!
2024-11-18
Author: Sophie
Introduction
In a groundbreaking study led by University of Toronto researchers, a new computational method has emerged that promises to transform our understanding of how chromosomes are organized within human cells. This research could be a game-changer for precision medicine, potentially unlocking new insights into how the reorganization of chromosomes contributes to disease progression.
Collaboration and Focus
Professor Samin Aref from the Faculty of Engineering collaborated with Professor Philipp Maass of the Temerty Faculty of Medicine’s Department of Molecular Genetics and the SickKids Research Institute. Their team focused on unraveling the complex structure of human chromosomes, the DNA strands packed with essential genetic information.
Historical Context
Historically, changes or abnormalities in chromosome structure have been associated with various diseases, including cancer. However, until now, scientists have lacked a comprehensive understanding of how these chromosomes are arranged within the nucleus of a cell. To bridge this gap, the team delved into the interactions between different chromosome parts to hypothesize their organization, and they subsequently validated their hypotheses through computational analysis and innovative imaging techniques.
Introduction of Signature Method
The team's findings, published in Nature Communications, introduce a novel computational approach called Signature, which leverages machine learning to unveil previously unknown patterns in the human genome. This method could open doors to understanding the genomic basis of numerous diseases.
Limitations of Traditional Methods
Traditional imaging methods, such as microscopy, have limitations, as they can only visualize one cell at a time—an inefficient and labor-intensive process. "You can label chromosomes and visualize their organization, but until now, identifying the specific genomic regions where these interactions occur was a challenge," explains Maass.
Innovative Data Analysis
The researchers cleverly combined the strengths of imaging with high-throughput chromosome conformation capture (Hi-C) datasets, which allowed them to analyze genomic data on an unprecedented scale—up to billions of sequencing reads from 62 different datasets, each containing over 3.8 million potential interactions.
Significance of Machine Learning
"Genetic data is inherently correlated and complex; hence machine learning is crucial for discerning meaningful patterns," notes Milad Mokhtaridoost, the study's lead author. The team applied two machine learning techniques: supervised learning, which relies on existing knowledge of what to look for, and unsupervised learning, which explores the data without preconceived notions.
Breakthrough Findings
Their breakthrough uncovered significant patterns regarding how chromosomes interact, providing initial results through the supervised method and confirming findings with unsupervised techniques. The interdisciplinary nature of the project was essential, combining expertise in genetics, data science, machine learning, and microscopy to achieve these innovative results.
Future Directions
Now, the researchers plan to extend their methodology beyond healthy genomic data to explore cancer genomics. "Understanding how the genome is organized in healthy samples gives us a baseline to investigate the rearrangements seen in diseases and their impact," says Maass.
Conclusion
The implications of this research are profound—by identifying how chromosomal contacts are altered in disease states, there is potential to pioneer new prevention strategies and therapeutic methods. This promising intersection of machine learning and molecular genetics is set to pave the way for significant advancements in personalized medicine, propelling us closer to more effective disease management. Stay tuned for what these scientific pioneers uncover next!