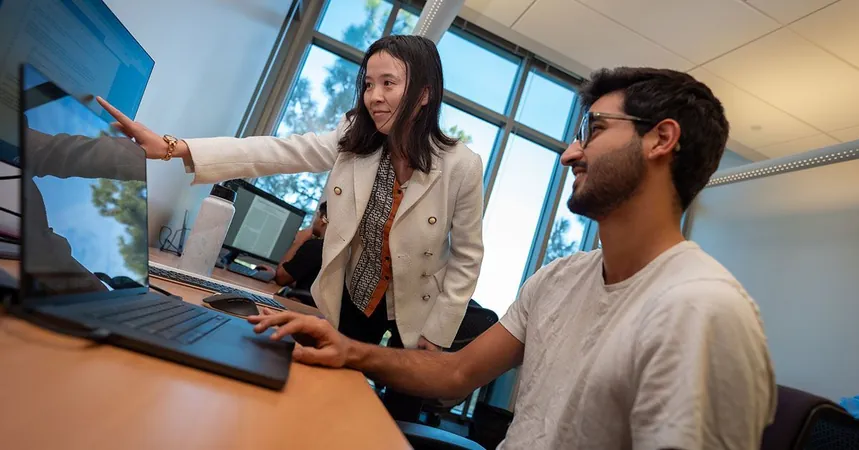
Revolutionizing Climate Prediction: How Generative AI is Changing the Game
2024-12-02
Author: Emily
Revolutionizing Climate Prediction: How Generative AI is Changing the Game
In an exciting development for climate science, computer scientists from universities in Seattle and San Diego have made a breakthrough by leveraging generative AI to enhance climate modeling. Their innovative model, known as Spherical DYffusion, utilizes advanced algorithms from generative AI tools, akin to those powering DallE, combined with physical data to predict climate behaviors more efficiently than ever imagined.
This cutting-edge model offers the incredible capability to forecast climate patterns over the next century in just 25 hours—a task that currently takes traditional models several weeks to complete. The implications are astounding: while existing models necessitate the use of supercomputers, Spherical DYffusion can run on standard GPU clusters available in research labs, making this technology more accessible to researchers worldwide.
The researchers make a bold claim: "Data-driven deep learning models are on the verge of transforming global weather and climate modeling." This statement reflects a growing trend, as scientists increasingly recognize the potential of AI to provide timely and critical predictions in an era where climate change poses a billion-dollar question for economies and ecosystems alike.
The team is set to unveil their findings at the highly-anticipated NeurIPS conference, running from December 9 to 15 in Vancouver, Canada, where experts and enthusiasts alike will gather to discuss advancements in AI and machine learning.
Generating accurate climate simulations has traditionally been an expensive endeavor, which restricts scientific and policy-making exploration to only a narrow set of scenarios. The team's groundbreaking discovery lies in employing generative AI models, notably diffusion models, for ensemble climate projections. By integrating these with a Spherical Neural Operator—a neural network adept at processing spherical data—they’ve created a model that initiates with foundational climate knowledge and iterates through learned transformations to predict future climate trends.
One of the major perks of this approach is efficiency. The researchers emphasize that while conventional diffusion models can produce realistic predictions, they often fall short in terms of speed and require significant computational resources. The new model strikes a balance, achieving near-comparable accuracy at a fraction of the computational cost, which may open doors to extensive climate scenario testing previously deemed impossible.
However, the team acknowledges that their work is not without challenges. Future iterations of Spherical DYffusion will aim to include more complex elements in their simulations, with plans to model atmospheric responses to rising CO2 levels—a critical factor in understanding climate change impacts.
Rose Yu, a prominent figure from UC San Diego's Department of Computer Science and Engineering, stated, "We emulated the atmosphere, which is one of the most important elements in a climate model," highlighting the foundational work that has gone into this research.
This remarkable collaboration traces back to an internship by one of Yu’s Ph.D. students, Salva Ruhling Cachay, at the Allen Institute for AI, showcasing the dynamic synergy between academia and artificial intelligence research.
In summary, while the battle against climate change intensifies, innovations like Spherical DYffusion may usher in a new era of climate modeling that could fundamentally alter how we predict and respond to our planet’s evolving climate dynamics. Researchers are optimistic that these advancements will lead to actionable insights for policymakers and scientists alike, providing the tools needed to tackle one of humanity’s largest challenges in modern history.