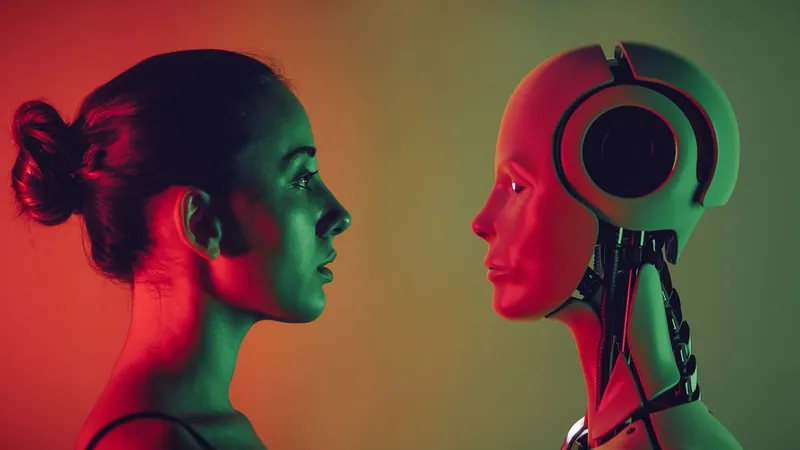
Shocking Differences Between Human and AI Thinking Revealed: What This Means for the Future!
2025-04-01
Author: Michael
Recent research has unveiled startling differences in the cognitive processes of humans versus artificial intelligence (AI), with potential consequences that society may not be ready for. While it's long been established that AI does not "think" like a human, new findings suggest that these distinctions could significantly impact how AI makes decisions in real-world scenarios.
Published in February 2025 in the journal Transactions on Machine Learning Research, this groundbreaking study investigates the capabilities of large language models (LLMs) to form and understand analogies. The research highlights that while humans excel in both basic letter-string analogies and digital matrix problems, AI systems experience a dramatic decline in performance on these tasks.
For instance, the study examined how well humans and AI could tackle story-based analogy problems, uncovering that AI models showed vulnerabilities to answer-order effects. This means that the sequence in which answers are presented can alter AI responses, indicating a lack of cognitive reliability. AI systems were also found to be prone to paraphrasing, further exhibiting their limits in understanding nuanced meaning.
The core finding revealed that AI models are deficient in “zero-shot” learning—the ability to deduce the context of new, untrained categories based on examples. This is a critical function for effective reasoning, showcasing a significant cognitive gap compared to humans.
Martha Lewis, a co-author of the study and an assistant professor specializing in neurosymbolic AI at the University of Amsterdam, presented a striking example to illustrate this deficiency. She noted that while humans intuitively eliminate repeated elements in analogy tasks, AI models like GPT-4 struggle to identify such patterns accurately. For instance, when faced with the analogy "if abbcd goes to abcd, what does ijkkl go to?" humans often respond with 'ijkl', yet AI frequently fails to reach the same conclusion.
This discrepancy isn't just an academic concern; it has tangible implications. As AI becomes increasingly embedded in sectors like law—where it aids in research, interprets case law, and assists in sentencing recommendations—its limited capacity to recognize subtle legal precedents could lead to significant misjudgments. Such flaws in AI reasoning could ultimately affect justice outcomes for individuals.
Lewis emphasizes that identifying patterns is not the same as understanding and abstracting from them. AI's dependency on extensive training data can help it spot trends, but without the capability to generalize knowledge, its cognitive function remains superficial.
As AI systems become more integrated into everyday decision-making, the study underscores an urgent need to assess these technologies for robustness, not just accuracy. This distinction is crucial; simply relying on AI for complex problem-solving could lead to unforeseen consequences, sparking discussions about the ethical integration of AI into critical life domains. The urgency for rigorous evaluation grows as our reliance on these technologies deepens.
What does this mean for the future of AI in our lives? As experts warn about the repercussions of these cognitive limitations, it's clear that society must tread carefully in adopting AI without fully understanding its boundaries—including how it thinks, or fails to think, like us.