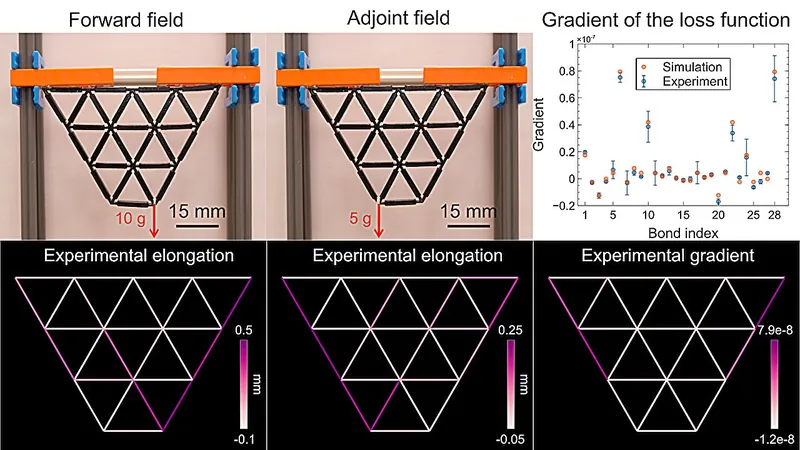
The Future of Learning Machines: Unlocking Materials That Can Adapt and Evolve
2024-12-09
Author: Liam
Machine learning has often been viewed as a strictly digital domain, driven by complex algorithms and high-powered computers mimicking human-like cognitive processes. Surprisingly, emerging research reveals that the foundations of machine learning can also rest in the physical realm, particularly within the realm of mechanical systems. A pioneering study from physicists at the University of Michigan highlights this transformative potential, ushering in a new era where materials might learn and adapt just like we do.
The innovative team led by Shuaifeng Li and Xiaoming Mao has engineered an algorithm that lays out a mathematical framework designed for how learning can occur in mechanical neural networks (MNNs). “We’re discovering that materials can independently learn tasks and perform computations,” said Li, shedding light on the project's ground-breaking implications.
Their research demonstrates the capability of these materials to be “trained” to accomplish various tasks, including differentiating between iris plant species. This pioneering approach heralds a future where materials could adapt structures, such as airplane wings that optimize for different aerodynamic conditions autonomously, without human intervention or digital assistance.
While this promising future is still on the horizon, the current findings present immediate insights that could inspire research across various scientific fields. Li noted that their new algorithm, grounded in established backpropagation techniques, could also propel inquiries into the learning mechanisms of living systems. “We are witnessing the successful application of backpropagation in diverse physical contexts,” Li pointed out, indicating potential interest for biologists studying human and animal neural processes.
MNNs Simplified
The integration of physical objects into computational processes has long been a topic of discussion. However, the focus on mechanical neural networks has gained momentum alongside recent advancements in artificial intelligence (AI). As AI technologies proliferate, with millions relying on systems like ChatGPT for tasks ranging from email drafting to travel planning, there is an increasing interest in how similar methodologies can be applied to materials.
To illustrate, the operations of a chatbot involve users inputting commands that an artificial neural network processes, ultimately generating responses based on its learned experiences. Correspondingly, mechanical neural networks operate under analogous principles. In Li and Mao's study, the input was applied force on a material, which served as the processing entity, while the output manifested in the physical deformation of that material.
The materials used were sophisticated, rubbery 3D-printed lattices composed of triangular shapes forming trapezoidal structures. These lattices learn by modulating their stiffness or flexibility in specific segments, paving the way for future applications where materials autonomously adjust their characteristics.
For MNNs to reach their futuristic applications—like self-tuning airplane wings—it's essential for them to adapt these segments independently. Although research in this area is underway, ready-to-use solutions are not yet commercially available. Currently, Li simulates behaviors by altering the thickness of material segments to achieve desired reactions. Their algorithm represents a key advancement in guiding these materials to self-adjust.
Teaching Your Mechanical Neural Network
While the mathematics surrounding backpropagation can be intricate, its core concept is straightforward. To begin the training process, one must establish the input and desired output. By applying the input and analyzing discrepancies between actual and desired outcomes, the system iteratively refines itself, guided by a mathematical operator called a gradient.
Li explains, “If you know precisely what you’re investigating, our MNNs can unveil that information effortlessly." High-tech tools, including cameras and specialized coding, supplemented their research efforts in obtaining this data, enhancing efficiency and convenience.
Imagine a situation where a lattice has uniform segments. If a weight is suspended from a central node, the force would symmetrically distribute, causing equal downward movement on either side. However, if the team aimed to create a lattice that maximized asymmetrical responses, they could leverage their algorithm to achieve unparalleled results, mirroring neuronal behavior in biological systems.
The researchers also collected extensive datasets of input forces to refine their MNNs, paralleling traditional machine learning protocols. One notable experiment involved analyzing iris plants, where various force inputs corresponded to different petal and leaf sizes. The trained lattice could then accurately identify unfamiliar plant species based on its training data.
Excitingly, Li is pushing the boundaries of this technology by exploring the possibilities of MNNs capable of processing sound waves. “We can encode an even richer tapestry of information into the inputs,” he stated. Sound waves allow for multiple parameters—amplitude, frequency, and phase—all of which can convey data, expanding the horizons of this innovative research.
Conclusion: The Learning Machines of Tomorrow
The burgeoning world of mechanical neural networks signifies a pivotal shift in how we conceptualize learning beyond the digital sphere. As researchers at institutions like the University of Michigan continue to unlock the secrets of materials that can learn, the implications could extend far beyond academic exploration, revolutionizing industries and scientific understanding. The future of intelligent adaptive materials is not just on the horizon; it's beginning to take shape right before our eyes!