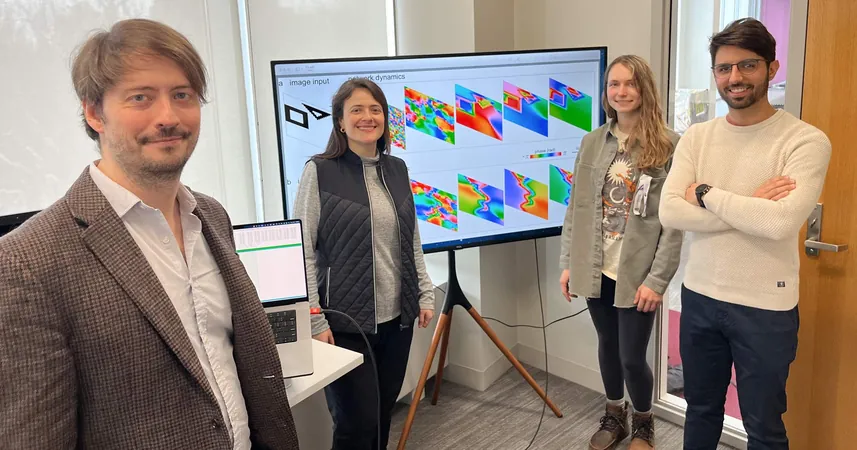
Unlocking the Secrets of AI: Researchers Use Math to Illuminate Machine Learning Mysteries!
2025-01-13
Author: Jacob
In a groundbreaking development, researchers from Western have unveiled a cutting-edge mathematical technique aimed at demystifying the decision-making processes of neural networks, a crucial component in the burgeoning field of machine learning. This innovative approach has ignited discussions about the transparency of AI, which has increasingly come under scrutiny as its applications range from digital assistants like Siri and ChatGPT to life-saving medical imaging and autonomous vehicles.
Neural networks, often likened to "black boxes," have earned this reputation due to their complex and opaque decision-making mechanisms. "We create neural networks that can perform specific tasks while also solving the equations that govern their activity," explains Lyle Muller, a mathematics professor and the director of Western’s Fields Lab for Network Science. "Our mathematical solution allows us to ‘open the black box’ and gain insights into how these networks operate."
The research, conducted in collaboration with international experts including Max Welling from the University of Amsterdam, was published in the esteemed journal PNAS. The team’s pioneering work centers on image segmentation—a foundational process in computer vision where machine learning systems identify and separate different elements within an image.
Starting with basic geometric shapes such as squares and triangles, the Western team, which included postdoctoral researchers Luisa Liboni and Roberto Budzinski, along with graduate student Alex Busch, successfully trained a neural network to perform image segmentation. Employing their innovative mathematical framework, the researchers assessed how the network executed this task and were astounded to find that it could not only handle basic shapes but also interpret complex natural images, such as a polar bear in a snowy landscape or a wild bird.
By simplifying the segmentation process, the researchers were able to create a network that demonstrated superior flexibility and performance on previously unseen inputs. "This discovery marks just the beginning," Muller notes, hinting at broader implications for machine learning. "Our mathematical insights could have far-reaching applications beyond this initial study."
The influence of this research ripples across various domains. In a related study scheduled for publication in *Communications Physics* in 2024, Muller and his colleagues developed a similarly "explainable" neural network capable of executing diverse tasks, from logical reasoning to secure communication protocols. Moreover, in collaboration with Professor Wataru Inoue and his team at the Schulich School of Medicine & Dentistry, they made a pioneering move by connecting their neural network to a living brain cell, creating a hybrid system that fuses artificial and biological intelligence.
"This fundamental understanding is vital as we advance towards more sophisticated AI systems that are reliable and trustworthy," adds Budzinski, providing a glimpse into the potential future of artificial intelligence that could enhance our daily lives while ensuring transparency and accountability.
This remarkable research not only aims to revolutionize how we interact with AI but also sets the stage for the next generation of machines that we can trust—leading us closer to a future where technology and humanity thrive together!