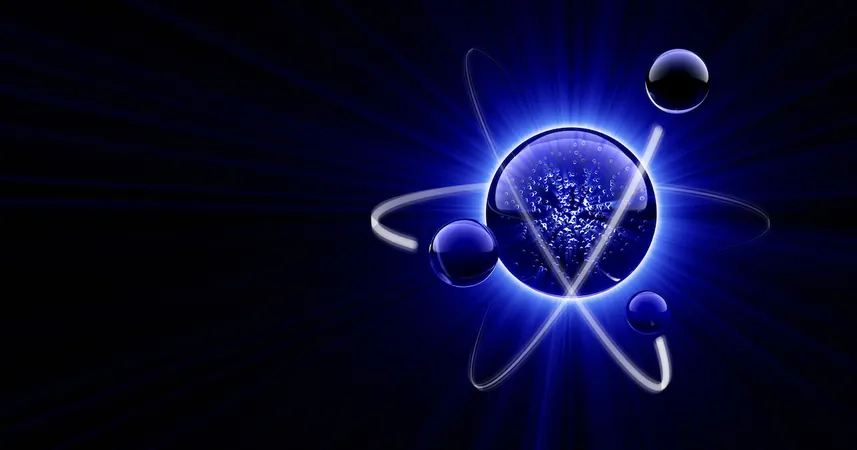
Unveiling the Mysteries of AI: Anthropic’s Revolutionary 'AI Microscope'
2025-04-12
Author: Charlotte
Delving Deeper into AI Mechanics
Anthropic has recently released two groundbreaking papers that aim to illuminate the enigmatic processes within large language models (LLMs). These studies focus on how interpretable concepts can be pinpointed and connected to the computational "circuits" responsible for transforming these concepts into coherent language, while also examining critical behaviors of Claude Haiku 3.5, including hallucinations and advanced planning.
The Complexity of AI Understanding
Despite their impressive capabilities, the inner workings of large language models remain largely opaque. This lack of clarity complicates efforts to explain how these models tackle challenges and generate responses. Anthropic seeks to peel back these layers, introducing their innovative tool—dubbed the 'AI Microscope'—to decipher the intricate reasoning that fuels LLMs.
Inspired by Neuroscience
Drawing inspiration from neuroscience's exploration of cognitive processes, the AI Microscope enables researchers to identify activity patterns and information flows within AI systems. In simplified terms, this involves substituting a model under investigation with a 'replacement model'—one that utilizes sparsely-active features representing understandable concepts. For instance, a feature might activate just before the model generates a state capital.
Crafting the Perfect Replacement Model
Of course, this replacement model may not mirror the original model's outputs exactly. To mitigate this, researchers develop a localized version tailored to specific prompts, integrating error terms and fixed attention patterns. This ensures that the replacement model closely aligns with the original outputs while maximizing interpretable features.
The Power of Attribution Graphs
To track the journey of features from an initial prompt to the final response, Anthropic researchers construct an attribution graph. This graph meticulously prunes away irrelevant features to reveal the significant ones contributing to the output, significantly enhancing our understanding of AI thought processes.
Revolutionary Findings About Multilingual Capabilities
Using this novel approach, Anthropic has uncovered intriguing insights, particularly regarding multilingual functions. Their studies suggest the existence of a universal language that Claude utilizes to conceptualize ideas before translating them into specific languages. When prompted for the "opposite of small" in various languages, identical core features for these contrasting concepts activate, leading to a coherent concept of largeness that gets articulated in the relevant language.
Planning and Creativity in AI Outputs
Contrary to the popular belief that LLMs construct sentences without much foresight, Anthropic's work challenges this notion. When analyzing Claude's rhyming abilities, researchers found evidence of advanced planning—prior to penning a second line, Claude "thought" about suitable rhyming words, illustrating a level of creativity and anticipatory structuring.
Understanding AI Hallucinations
Exploring the phenomenon of AI hallucinations—instances where the model generates inaccurate information—was another focal point. Researchers concluded that such hallucinations are somewhat intrinsic to the model's functioning; they must perpetually generate predictions, which necessitates a balancing act between recognizing known entities and acknowledging uncertainty. When a recognized name triggers a response without sufficient knowledge, the model might mistakenly generate incorrect information, leading to confabulation.
Future Directions in AI Interpretability
In addition to addressing hallucinations, Anthropic's investigations encompass mental math, multi-step reasoning, and the development of coherent thought chains. Their ultimate goal is to enhance interpretability in AI, creating tools that clarify how models derive conclusions and ensuring alignment with human values. However, it's essential to note that this pioneering effort currently captures only a fraction of the model's computations and is limited to small prompts. Stay tuned for more updates on advancements in AI interpretability as this field rapidly evolves!