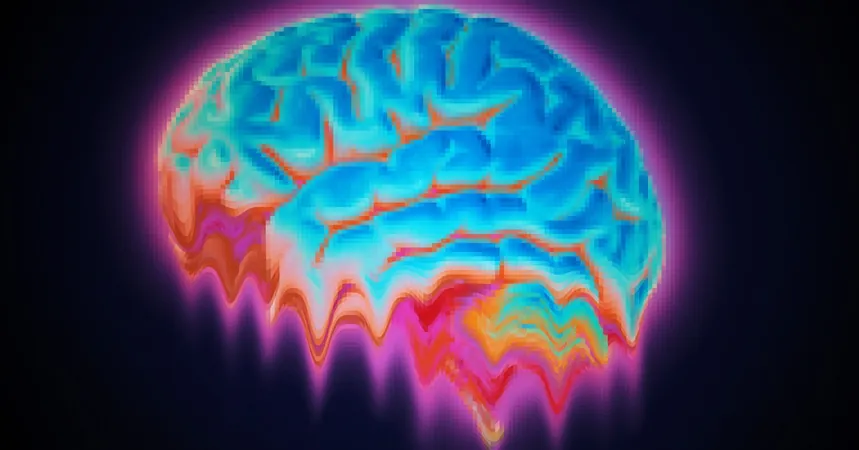
Apple Engineers Expose Shocking Fragility of AI 'Reasoning'
2024-10-15
Author: Yan
Apple Engineers Expose Shocking Fragility of AI 'Reasoning'
In a groundbreaking study, Apple engineers have revealed that the touted "reasoning" abilities of advanced artificial intelligence models, such as those from OpenAI and Google, may be far more fragile than previously believed. The research, led by six experts from Apple, suggests that while these models can perform complex mathematical tasks, their method of problem-solving is not as reliable as it appears.
The Illusion of Reasoning: A Closer Look
The paper, titled "GSM-Symbolic: Understanding the Limitations of Mathematical Reasoning in Large Language Models," presents a stark critique of the current capabilities of large language models (LLMs). By modifying a popular benchmark set of grade-school math problems, the researchers assessed how small changes in the content—such as swapping names and numbers—affected model performance. Shockingly, the results indicated that even minor alterations led to significant declines in accuracy.
Across more than 20 state-of-the-art models tested on this revised benchmark, the average accuracy dropped between 0.3% and 9.2%. This inconsistency raises critical concerns about the core approach these models utilize: probabilistic pattern matching, rather than authentic logical reasoning. The researchers argue that these models essentially mimic the reasoning steps they were trained on, rather than genuinely grasping the underlying mathematics.
Mind-Blowing Results from GSM-Symbolic
What’s particularly perplexing is that while the structure of the mathematical queries remained the same, the models’ accuracy fluctuated wildly. During numerous trials, performance variance reached up to 15%, emphasizing a startling unreliability in how these AI systems handle reasoning.
To further test the models, the researchers introduced a version of the benchmark with trivial yet irrelevant details—dubbed “GSM-NoOp.” This manipulation resulted in catastrophic drops in accuracy, with declines ranging from 17.5% to as much as 65.7%. Such a performance collapse underscores the inability of LLMs to distill crucial information from complex prompts, showcasing a critical flaw in their programming.
The Implications for AI Development
These findings echo sentiments shared by AI experts, including Gary Marcus, who argue that true advancements in artificial intelligence will require models capable of integrating genuine symbol manipulation. Such capabilities would mirror the processes used in algebra and traditional computer programming—allowing machines to truly understand abstract representations rather than patching together responses based on similar patterns in their training data.
The research serves as a stark reminder that while AI can produce impressive results, it also harbors significant limitations. The so-called "illusion of understanding" becomes particularly evident when these models face unexpected scenarios or variations, leading to errors that a simple calculator would not make.
As we navigate the evolving landscape of AI, this revelation invites deeper reflection on how we train these systems and the true nature of their cognitive abilities. While current advancements in AI are remarkable, this research elucidates the fragility that lies beneath the surface, challenging the notion that LLMs possess true reasoning capabilities.
Stay tuned for further developments in AI technology as researchers continue to explore these critical issues!