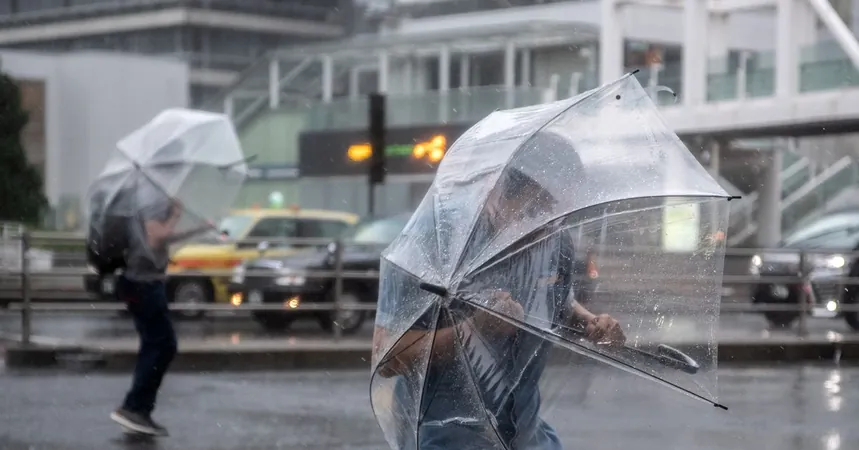
Google's Game-Changing AI Weather Model Could Revolutionize Forecasting!
2024-12-07
Author: Lok
Introduction
Google DeepMind has unveiled GenCast, an innovative AI weather prediction model that is making headlines for its impressive forecasting capabilities. Recent studies reveal that GenCast can outperform established weather models, showing up to 97.2% accuracy compared to the European Centre for Medium-Range Weather Forecasts' (ECMWF) ENS model based on data from 2019.
Significance of GenCast
Key researchers, including senior scientist Ilan Price, emphasize the significance of GenCast not only in providing more precise forecasts but also in potentially reshaping meteorological practices. "Weather is a critical factor in everyday life and remains one of science's most daunting challenges," says Price. "Our mission at Google DeepMind is to advance AI for the benefit of humanity, and GenCast could be a critical tool in achieving that."
How GenCast Works
GenCast gathers its intelligence from a rich dataset covering weather patterns from 1979 to 2018. Unlike traditional models that depend on complex calculations executed by powerful supercomputers, GenCast employs machine learning to identify weather patterns. This fundamental difference means GenCast is capable of delivering forecasts quickly—generating a 15-day prediction in just eight minutes, while traditional models like ENS can take hours.
Advancements in Cyclone Prediction
One standout feature of GenCast is its ability to enhance tropical cyclone predictions. In tests, it provided an additional 12 hours of advance warning for cyclone paths—a critical lead time that can help save lives and property. Besides cyclone tracking, GenCast also excels in predicting extreme weather events and wind power generation, extending forecasts up to 15 days out.
Comparison with Existing Models
However, it's noteworthy that GenCast was benchmarked against an older version of the ENS model. The latest version of ENS operates at higher resolutions, complicating direct performance comparisons. The current iteration of ENS has improved significantly since 2019, leading experts like ECMWF’s machine learning coordinator, Matt Chantry, to recommend continuous observation of how both models evolve.
Technical Specifications
In terms of technical specifications, GenCast operates on a 0.25-degree resolution, while the new ENS model runs at a 0.1-degree resolution, making it crucial to see if GenCast can match or exceed this benchmark in the future. Despite some limitations, Chantry notes that GenCast represents a substantial advancement in weather forecasting technology.
Sustainability Considerations
Price highlighted another critical advantage: sustainability. GenCast’s operational efficiency could mitigate the environmental concerns associated with energy consumption in AI data centers, which have been a growing issue for technology giants like Google. However, the total environmental impact of training such models remains under scrutiny.
Challenges Ahead
Yet, challenges lie ahead for GenCast. The model produces forecasts at 12-hour intervals, which may not provide the granularity needed for applications like renewable energy assessments. "For wind forecasts, frequent updates are essential," says Stephen Mullens, a meteorology professor who was not involved in the study. The meteorological community is still cautiously evaluating AI’s role in forecasting, as many professionals are trained in physics-based approaches.
Open-Source Availability
For those eager to explore this cutting-edge technology, DeepMind has made the GenCast model open-source, allowing forecasters to test and refine its capabilities. Price envisions a future where GenCast and similar AI applications work in tandem with traditional methods to create a more robust forecasting landscape.
Conclusion
As the debate continues regarding the best approach to weather forecasting, one thing is clear: GenCast is pushing the boundaries of what’s possible and has the potential to become a game-changer in the meteorological world.