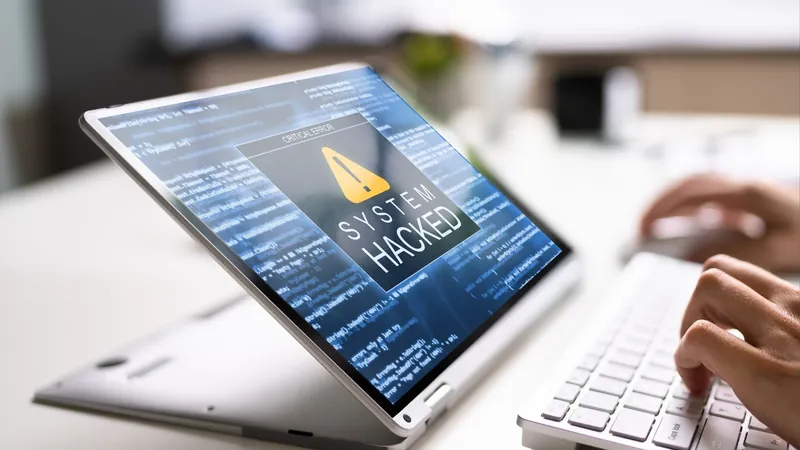
Alarming Security Flaws Discovered in Open-Source Machine Learning Systems: Are Your Data and Operations at Risk?
2024-12-22
Author: Ming
Recent revelations have sent shockwaves through the tech world as critical security vulnerabilities in popular open-source machine learning (ML) frameworks threaten sensitive data and operational integrity. A new report from JFrog highlights the urgent need to address these issues as ML technologies become increasingly ubiquitous across various industries.
The Sobering State of ML Security
With ML adoption skyrocketing, the security of these tools demands immediate attention. JFrog’s findings indicate a troubling trend, identifying 22 vulnerabilities across 15 different ML tools in just the past few months. The main concerns revolve around server-side components and escalating privileges within ML environments, leading to dire consequences where malicious actors could access sensitive files or compromise entire ML workflows.
One of the most significant discoveries pertains to Weave, a tool associated with Weights & Biases (W&B) that is instrumental in tracking and visualizing ML model metrics. The WANDB Weave Directory Traversal vulnerability (CVE-2024-7340) stems from inadequate input validation for file paths, allowing intruders to access crucial files like admin API keys. Such access can result in privilege escalation, endangering the integrity of the entire ML pipeline.
Multiple Tools at Risk
ZenML, another vital tool within the MLOps space, is also compromised. A critical vulnerability in ZenML Cloud’s access control mechanisms could enable attackers, even with minimal access rights, to escalate their privileges. This flaw puts confidential data such as secrets and model files at significant risk, potentially allowing for the manipulation or sabotage of production environments reliant on these pipelines.
The threat landscape expands with the Deep Lake database, utilized for AI applications, which presents a severe risk through its Deep Lake Command Injection vulnerability (CVE-2024-6507). This flaw arises from inadequate command sanitization, empowering attackers to execute arbitrary commands that can jeopardize both the database and its connected applications.
Moreover, Vanna AI, known for its natural language SQL query generation capabilities, is vulnerable due to the Vanna.AI Prompt Injection (CVE-2024-5565). This vulnerability lets attackers embed malicious code into SQL prompts, leading to potential remote code execution. The implications are grave, risking data theft and potentially manipulated visualizations.
Mage.AI, a platform designed for managing data pipelines, shows susceptibility to unauthorized shell access, file leaks, and path traversal issues, all of which can lead to profound breaches in data integrity and control.
Why This Matters Now More Than Ever
The alarming vulnerabilities unveiled by JFrog underscore a precarious gap in MLOps security. Many organizations still don’t incorporate AI/ML security into their overarching cybersecurity strategies, leaving them exposed to potential attacks. Not only could these weaknesses allow intruders to insert malicious code into models, but they could also lead to data theft or manipulation of ML outputs, resulting in chaos within affected systems.
As ML and AI technologies continue to revolutionize industries from healthcare to finance, securing these frameworks, alongside the data and models they rely on, has never been more critical. Organizations must prioritize and implement robust security practices to safeguard these transformative innovations. Failure to act now could lead to significant repercussions, including data breaches and operational catastrophes.
Stay informed and vigilant – your data could be just a vulnerability away from danger!