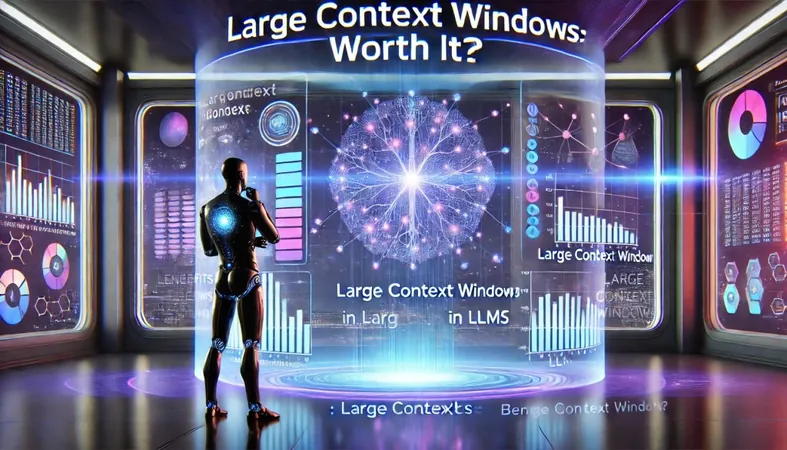
Beyond the Token Limit: The Future of Large Language Models in Business
2025-04-12
Author: Arjun
The Great Context Debate in AI
The AI landscape is buzzing as tech giants race to push large language models (LLMs) past the million-token mark. With powerful models like MiniMax-Text-01 boasting capacities of 4 million tokens, and Gemini 1.5 Pro handling up to 2 million tokens at once, the implications for applications in coding, legal analysis, and research are enormous.
Central to this discussion is context length—essentially, how much text an AI can digest and remember in a single shot. Imagine a model that can process the equivalent of 10,000 pages of text all at once! This should theoretically improve understanding and reasoning, but does it translate into real business benefits?
The Hype vs. Reality of Large Context Windows
Big names like OpenAI, Google DeepMind, and MiniMax are in a competitive scramble to extend these context lengths, promising deeper comprehension and more reliable outputs. For businesses, this means that algorithms could potentially analyze entire contracts or summarize lengthy reports without losing the thread of context.
However, removing the need for techniques like chunking and retrieval-augmented generation (RAG) is not without its challenges. Can the increased memory really solve the 'needle-in-a-haystack' problem, where critical information is often overlooked within vast datasets?
Unlocking Efficiency with Larger Contexts
Larger context windows can boost accuracy, enhancing capabilities like cross-document compliance checks and medical literature comparisons. A recent Stanford study found that 128K-token models significantly reduced hallucination rates during complex analyses—a good sign for industries reliant on precision.
Nevertheless, early adopters are finding that these expansive models aren’t infallible. For instance, JPMorgan Chase's findings showed that context performance falters beyond certain limits, raising critical questions about whether this leap is genuinely beneficial or merely an expensive way to expand memory.
The Cost of Performance: RAG vs. Large Prompts
Choosing the right approach isn’t straightforward. RAG, which couples LLMs with external databases, allows for efficient information retrieval, minimizing costs and memory use. Large prompts can deliver a one-stop analysis but hit hard on budgeting due to intensive computational requirements.
The dilemma is clear: when should companies opt for massive context models versus RAG-based retrieval? Some scenarios favor deep analysis of documents, while others thrive on dynamic queries where speed and cost matter more.
Navigating Diminishing Returns
But as we scale up context, we face crucial limitations: latency, rising costs, and usability. More tokens processed often lead to slower responses, and making these models work efficiently can become prohibitively expensive.
While innovative techniques like Google’s Infini-attention are emerging to tackle these trade-offs, they come with trade-offs of their own, including potential information loss.
The Path Ahead: Hybrid Models for the Future
As we move forward, the path might not be about adopting the biggest models but rather leveraging hybrid systems that efficiently balance the scales between RAG and large prompts. Companies must critically assess their use cases, setting clear budget limits and distinguishing between complex reasoning needs and simpler tasks.
As industry experts like Yuri Kuratov highlight, expanding context without enhancing reasoning capabilities is futile—akin to building wider highways for cars that can’t navigate them. The future of AI must be about models that truly comprehend and communicate across expansive narratives.