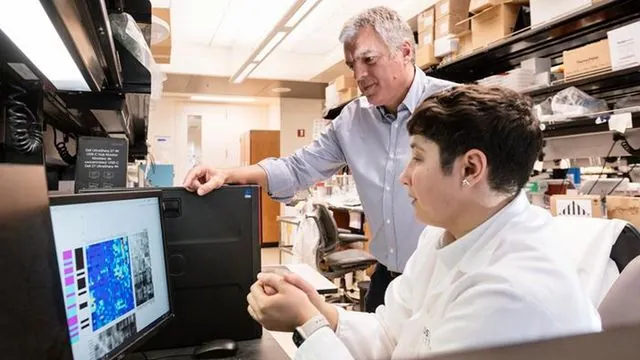
Breakthrough Machine Learning Tool Detects Early Alzheimer's Signs Before Symptoms Emerge!
2024-11-27
Author: Rajesh
A groundbreaking study reveals that subtle signs of Alzheimer’s disease can often manifest decades before a formal diagnosis—frequently in the form of irregular behaviors indicating early-stage brain dysfunction.
The challenge has long been identifying and measuring these slight behavioral changes scientifically, even in studies involving mice.
Published in *Cell Reports*, researchers from Gladstone Institutes have developed an innovative video-based machine learning tool that can detect otherwise undetectable signs of early Alzheimer’s in specially engineered mice.
Their research marks a significant advancement in the quest to identify neurological diseases sooner and track their progression over time.
According to Gladstone investigator Jorge Palop, PhD, the senior author of the study, 'We’ve demonstrated how machine learning can transform our analysis of behaviors signaling early abnormalities in brain function. This opens the door to a more profound understanding of devastating brain disorders and their beginnings.'
To analyze the behavior of mice exploring a designated space, the team used a machine learning platform called VAME, which stands for Variational Animal Motion Embedding.
This open-source tool captured subtle behavioral patterns that traditional observation methods may have missed, allowing for a deeper understanding of the animals' conditions.
Revolutionizing Behavioral Analysis
VAME’s advanced deep learning capabilities differ significantly from conventional behavioral tests in mice, which typically center around predefined tasks.
This limitation restricts the ability to capture the full spectrum of spontaneous changes induced by neurological diseases, especially in their early stages.
In their work, the Gladstone team evaluated two types of mice modeled to exhibit different Alzheimer’s traits.
They observed that the machine learning tool successfully revealed a striking increase in 'disorganized behavior' as the mice aged.
Notable changes included unusual behavior patterns and increased transitions between various activities—indicators closely associated with deficits in memory and attention.
Stephanie Miller, PhD, a staff scientist at Gladstone and first author of the study, envisions broader applications: 'Similar machine learning techniques could one day assist in studying spontaneous behaviors in humans, potentially allowing for early diagnoses of neurological diseases.
With just smartphone-quality video, VAME could revolutionize patient assessments in clinics and homes alike.'
Potential Treatment Insights
In an additional phase of their study, the Gladstone team employed VAME to assess whether a potential therapeutic approach for Alzheimer’s could mitigate disorganized mouse behavior.
Building on previous work by colleague Katerina Akassoglou, PhD, who discovered that a blood-clotting protein known as fibrin induces toxic effects when it enters the brain, the researchers sought to block fibrin's harmful inflammation.
By genetically inhibiting fibrin's inflammatory impact, the team successfully reduced the abnormal behaviors associated with Alzheimer’s in mice.
Akassoglou expressed optimism regarding the findings: 'Seeing how blocking fibrin’s inflammatory role lessened almost all spontaneous behavioral changes in Alzheimer’s mice is very encouraging.
It reinforces that fibrin and the resulting neuroinflammation are significant contributors to the disease.'
Miller and Palop, along with other Gladstone teams focusing on neurological diseases, are now collaborating to implement VAME technology in various behavioral studies.
Miller emphasizes the potential impact: 'By making this tool and similar methodologies more accessible to biologists and clinicians, we aim to accelerate the development of effective new treatments.'