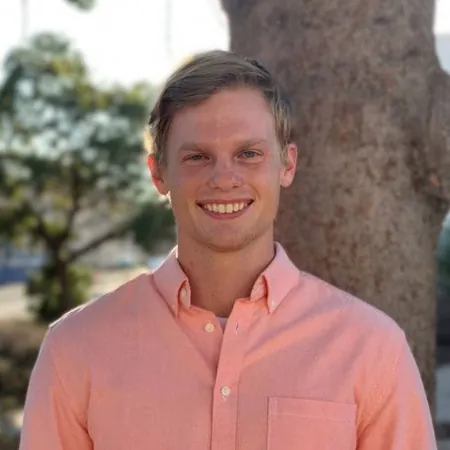
Groundbreaking Machine Learning Study Unveils Key Drivers of Social Isolation in Schizophrenia
2024-10-10
Author: Daniel
Groundbreaking Machine Learning Study Unveils Key Drivers of Social Isolation in Schizophrenia
Recent findings from a groundbreaking study utilizing machine learning have revealed critical insights into the factors contributing to social isolation and loneliness in individuals with schizophrenia. Research indicates that social anhedonia—a reduced ability to experience pleasure from social interactions—plays a significant role, while nonsocial cognition appears to elucidate unique variations of social isolation in this population.
The study, partly led by Dr. Samuel J. Abplanalp from UCLA’s Jane and Terry Semel Institute for Neuroscience and Human Behavior, employed advanced regression-based machine learning methods, specifically the Least Absolute Shrinkage and Selection Operator (LASSO). This innovative approach allowed researchers to analyze a multitude of interrelationships among psychological variables that influence mental health.
Dr. Abplanalp stated, “We utilized LASSO regression to assess how elements such as social cognition, nonsocial cognition, depression, social anhedonia, and social avoidance motivation correlate with social isolation and loneliness across various psychiatric samples.” This analysis encompassed individuals with schizophrenia, bipolar disorder, and a community comparison group enriched for social isolation.
Study Overview and Design
The research analyzed outcomes from 72 outpatients diagnosed with schizophrenia, 48 from bipolar disorder, and 151 individuals from the general community, all of whom participated in a larger initiative aimed at understanding the psychological dimensions of social disconnection. The participants were recruited from various outpatient clinics, including the Veterans Affairs Greater Los Angeles Healthcare System. Accurate diagnoses were confirmed using the Structured Clinical Interview for DSM-5.
The participants were selected based on stringent criteria to ensure clinical stability, evidenced by no hospitalizations in the preceding three months and no recent changes in medications. Additionally, targeted advertisements were utilized to recruit individuals experiencing significant social isolation, which highlighted feelings of minimal family contact and lack of engagement in solo activities.
Key Findings and Implications
The study's findings, based on machine learning techniques, revealed that levels of social isolation were comparable across the schizophrenia, bipolar disorder, and community samples, with no statistically significant differences noted. Notably, 27 individuals from the community sample were identified as having personality disorders—including borderline, avoidant, and schizotypal disorders.
Among participants, the predictive model for social isolation within the schizophrenia group accounted for 14% of the variance, while loneliness was attributed to 21% of the variance. Importantly, social anhedonia and nonsocial cognition were identified as significant predictors for social isolation in the schizophrenia cohort.
The study authors emphasized, “Social isolation and loneliness pose serious public health risks. Our research highlights social anhedonia as a transdiagnostic factor associated with these issues across schizophrenia and bipolar disorder, as well as the community sample with heightened isolation.”
The implications of these findings are far-reaching, suggesting that targeted interventions aimed at enhancing social cognition and addressing social anhedonia could be crucial in reducing social isolation and enhancing the overall well-being of individuals struggling with these psychiatric conditions. As mental health continues to gain prominence in public discourse, understanding these dynamics through innovative research methods marks a significant step forward in combating social isolation in vulnerable populations.