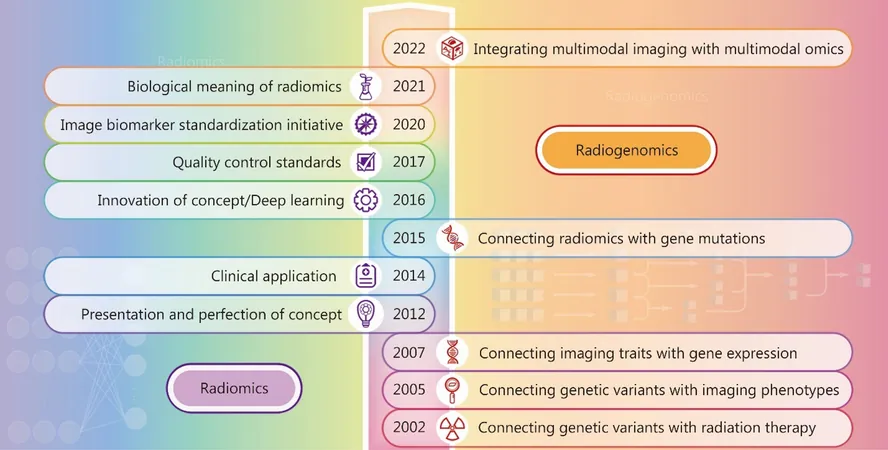
Radiomics and Radiogenomics: Transforming Ovarian Cancer Diagnosis and Prognostication
2024-12-14
Author: Daniel
Radiomics and Radiogenomics: Transforming Ovarian Cancer Diagnosis and Prognostication
Ovarian cancer (OC) remains one of the most challenging malignancies for clinicians due to its complex pathology and varied patient responses to treatment. However, recent advancements in radiomics and radiogenomics are paving the way for revolutionary improvements in diagnosis and prognosis prediction. This article delves into how these innovative approaches are reshaping the landscape of ovarian cancer management.
The Power of Radiomics in Diagnosis
Radiomics involves extracting a multitude of quantitative data from medical images, thus allowing for deeper insights into tumor characteristics. The precise classification of ovarian masses is crucial for tailoring optimal treatment strategies, whether that involves conservative management of benign lesions or aggressive interventions for malignant cases. Traditional imaging techniques often fall short due to the morphological similarities between benign and malignant tumors, underscoring the importance of refined diagnostic tools.
Several studies have demonstrated the effectiveness of radiomic analysis. For instance, research by Faschingbauer et al. showed that texture features derived from ultrasound images could significantly outperform less experienced physicians in diagnosing ovarian lesions. Similarly, advancements in artificial intelligence (AI) have led to the development of deep learning models capable of distinguishing benign from malignant tumors with remarkable accuracy.
A notable focus is on borderline ovarian tumors (BOTs), which have distinct characteristics and require careful differentiation from both benign and malignant ovarian tumors. Liu et al. created a groundbreaking model that achieved perfect diagnostic accuracy using 3D MRI features, emphasizing the importance of technological integration in clinical practice.
Advancements in Subtyping Ovarian Cancer
The subtyping of epithelial ovarian cancer (EOC) is equally critical, particularly in tailoring treatment regimens. Radiomics has been shown to enhance the accuracy of traditional diagnostic approaches by delineating aggressive tumor behaviors, thus enabling better therapy planning. Type I and Type II EOC each exhibit unique biological behaviors and responses to treatment - a vital distinction that can influence patient outcomes.
Studies incorporating varied radiomic features from MRI and CT scans have unveiled the potential of these models to outperform conventional methods in accurately identifying tumor subtypes. For instance, various models have shown promising results in distinguishing between high-grade serous ovarian cancers (HGSOC) and non-HGSOC, further refining treatment pathways such as neoadjuvant chemotherapy and surgical decision-making.
Predicting Outcomes: Survival and Recurrence
The inherent heterogeneity of EOC means that patient prognosis can vary dramatically, even among those with similar clinical stages. Radiomics possesses the potential not only to predict survival rates but also to identify patients at high risk of recurrence. Multiple studies have utilized radiomic signatures to develop nomograms that effectively stratify patients into risk categories, thus informing treatment intensity and follow-up strategies.
Emerging models based on machine learning and statistical analyses have demonstrated high accuracy in predicting outcomes such as progression-free survival (PFS) and overall survival (OS), leading to improvements in personalized treatment planning. As these models evolve, their clinical applications are poised to enhance patient management significantly.
Radiogenomics: A New Frontier
Combining radiomics with genomic data—known as radiogenomics—offers exciting possibilities for identifying genetic mutations and characteristics that drive individual tumor behavior. For instance, odynamic imaging features have been associated with BRCA mutations, which are critical for informing patients about potential responses to targeted therapies like PARP inhibitors.
The drive towards integrating imaging features with genetic data epitomizes the future of personalized medicine in ovarian cancer, where treatment regimens are tailored not just to tumor characteristics but also to the genetic makeup of individual patients.
Looking Ahead
While the current findings underscore the promise of radiomics and radiogenomics in revolutionizing OC management, ongoing research is essential to validate and refine these models across diverse populations and clinical settings. As more data become available, these approaches have the potential to dramatically improve ovarian cancer diagnosis, prognostication, and ultimately, patient outcomes.
In conclusion, the integration of radiomics and radiogenomics in clinical practice represents a significant leap forward in the fight against ovarian cancer—promoting earlier detection, personalized treatment, and improved survival rates. With each study contributing to a burgeoning body of evidence, the future of ovarian cancer management is increasingly bright.