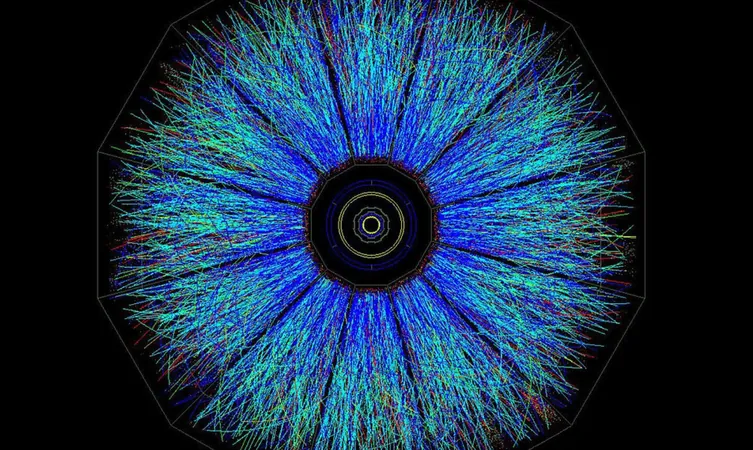
Revolutionary AI Boosts Gold Ion Collisions at Speeds Nearly Approaching Light
2024-12-11
Author: Sarah
Introduction
At Brookhaven National Laboratory (BNL), a cutting-edge research facility, scientists are pushing the boundaries of particle physics at the Relativistic Heavy Ion Collider (RHIC). Here, billions of gold ions zoom through an impressive 2.4-mile circular accelerator at speeds that approach the speed of light. These high-energy encounters, occurring thousands of times every second, break ions into fundamental particles, unlocking pivotal insights into the nature of the universe and its beginnings—especially in the milliseconds following the Big Bang.
Significance of RHIC
RHIC is renowned as the first facility capable of colliding heavy ions—atoms stripped of their electrons—providing data that is key to understanding cosmic events. However, orchestrating the precise collision of these beams, which travel in opposite directions, is no simple feat. It requires meticulously fine-tuning nine injector knobs, akin to juggling nine bowling pins while working in a physics lab. Historically, effectively managing these beams has relied heavily on the expertise of seasoned physicists.
Advancements in Beam Control
To enhance the beam intensity—essentially the density of gold ions within those beams—researchers from BNL, the Lawrence Berkeley National Laboratory, and Michigan State University have developed a groundbreaking machine learning algorithm. This innovative approach resembles adjusting a flashlight to produce a more intense beam of light. Their findings were recently showcased at the 15th International Particle Accelerator Conference in Nashville, Tennessee.
Expert Insights
“Colliders are complex and colossal machines,” stated Ji Qiang, a senior scientist at Berkeley Lab. “Our algorithm substantially reduces uncertainties and improves control over beams traveling at such extraordinary speeds.”
Boosting Performance Through Machine Learning
Machine learning algorithms work by processing large datasets to identify patterns and improve predictions. The RHIC's beam intensity, described by an incredibly complex mathematical function, has long eluded precise representation in equation form.
“Since the function embodying the beam characteristics is not established, we’re using data collection and our algorithm to decode it,” explained Sherry Li, a senior scientist on the research team. “Machine learning bridges this knowledge gap effectively.”
The team deployed their machine learning software, GPTune, to optimize nine control parameters for the RHIC's Electron Beam Ion Source (EBIS), which prepares the gold ions by stripping away electrons before they enter the collider. Utilizing gathered data and advanced statistical techniques, GPTune improved beam performance by making incremental adjustments.
Initially, beam intensity levels lingered below the desired threshold, but after evaluating 45 suggested configurations from GPTune, a breakthrough occurred—intensity levels began to recover. This pivotal moment was described by team member Xiaofeng Gu as the “most exciting” of the experiment, as subsequent adjustments led to a significant increase in ion intensity.
Ultimately, the algorithm achieved a remarkable 22% increase in beam intensity with further refinements. In a separate section, XF14, adjustments yielded even greater success, with averages increasing by a staggering 43%.
When both injection and extraction parameters were optimized simultaneously, the team was able to realize an impressive surge in beam intensity, achieving increases of 68-71% at the extraction location and 22-24% at the booster injection location.
A Bright Future Ahead
Looking to the future, researchers plan to implement the GPTune algorithm across additional beamlines at RHIC, which promises to dramatically enhance the overall luminosity of the detector. Moreover, the algorithm's potential is not limited to particle physics; it may also provide valuable insights in a range of scientific disciplines.
This innovative use of machine learning within particle physics signifies a pivotal advancement not only for RHIC but for the wider field, paving the way for deeper understanding of the universe and its underlying principles. Who knows what other mysteries this new technology might unravel next? Stay tuned as experts harness AI to power our quest for knowledge!