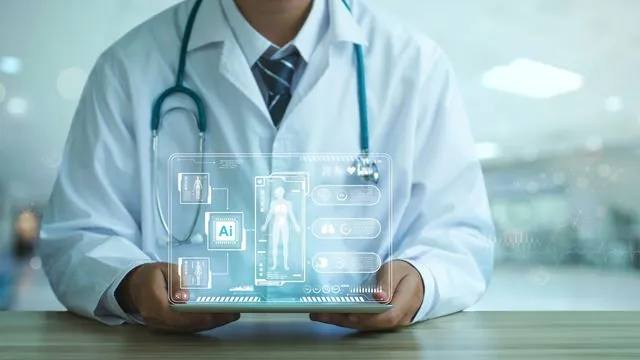
Revolutionary AI Enhances Organoid Development Efficiency in Medicine
2024-12-06
Author: Yu
Key Highlights:
AI Surpasses Expert Accuracy: The AI model provides superior predictions for organoid quality, offering a substantial improvement over traditional methods.
No Genetic Modifications Needed: The approach maintains the integrity of organoids by eliminating the need for genetic alterations, making it suitable for clinical applications.
Cost-Efficient and Time-Saving: This innovative method streamlines organoid selection, potentially reducing costs and increasing efficiency in research and medical applications.
Organoids in Biomedical Research
Organoids are poised to revolutionize biomedical research, offering solutions for personalized transplants, enhanced disease modeling, and refined drug testing. The recent study, published in Communications Biology, focuses specifically on hypothalamic-pituitary organoids, which emulate the pituitary gland's functions and produce adrenocorticotropic hormone (ACTH). This hormone plays a critical role in regulating stress, metabolism, and other vital functions. A deficiency in ACTH can lead to severe health issues.
Challenges in Organoid Development
Hidetaka Suga, an associate professor at Nagoya University's Graduate School of Medicine, emphasizes the potential of these organoids for treating ACTH deficiency in humans, based on promising animal studies. However, assessing organoid development has been challenging, primarily due to the sensitivity of these cell structures to minor environmental shifts, which can lead to variability in quality.
Early Protein Expression and AI Modeling
Researchers discovered that the expression of a protein known as RAX during the early stages of organoid development correlates with successful outcomes. Traditionally, evaluating this protein required genetic modifications for visualization, a method unsuitable for clinical applications.
In response to these limitations, the team collaborated with AI expert Hirohiko Niioka to develop a deep-learning model capable of analyzing organoid quality based solely on image data. By utilizing a combination of fluorescent and bright-field images, they effectively categorized organoids into three quality tiers. The best-performing ensemble model achieved a remarkable classification accuracy of 70%.
Validation of the AI Model
In a further test, the model demonstrated its utility by accurately predicting the quality of organoids without requiring any genetic modifications. The study revealed that those classified as high-quality by the AI also exhibited significant ACTH secretion after extended culture periods, thereby validating the model's predictive capabilities.
Future Prospects
This revolutionary use of deep-learning technology represents a first in the field, and the researchers are eager to refine the model using larger datasets. As Hidetaka Suga articulates, "With this model, we are on the verge of greatly improving our ability to quickly and efficiently select high-quality organoids for clinical applications."
In summary, as AI technology continues to evolve and integrate into biomedical research, the impact on organoid development and regenerative medicine is poised to be transformative, leading to substantial enhancements in patient care and innovative treatment solutions. This breakthrough not only exemplifies the potential of AI in science but also hints at a future where medical research is more efficient, accurate, and accessible.