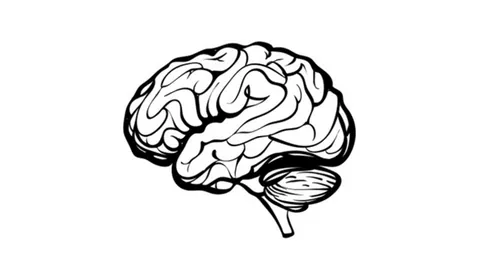
Revolutionary AI Tool Set to Transform Decision-Making in Treating Brain Metastases
2024-12-28
Author: John Tan
Introduction
A groundbreaking machine learning tool is changing the landscape of decision-making in stereotactic radiosurgery (SRS) for patients with small brain metastases. The focus of this innovative technology is to analyze critical factors like radiation dosage, patient demographics, and treatment-related variables, predicting the likelihood of local failure at 6 months, 1 year, and 2 years after treatment.
Challenges in Treatment
Brain metastases, particularly those measuring less than 2 cm, pose significant hurdles in achieving effective local control following SRS. Current treatment protocols often prescribe doses of 20 Gy, 22 Gy, or 24 Gy based solely on general clinical guidelines, which inadequately consider individual patient conditions.
AI-Driven Model for Local Failure Assessment
The introduction of this AI-driven model significantly enhances clinicians' ability to evaluate patients' local failure risks based on various parameters, including the prescribed dose, age, Karnofsky Performance Score (KPS), and treatment course. "Our goal is to scrutinize patient characteristics and treatment factors to accurately assess the risk of local failure for each dose level over time," stated Dr. Rupesh Kotecha, who oversees radiosurgery at Baptist Health Miami Cancer Institute. "To achieve this, we must analyze a vast amount of data beyond our conventional methods, with a solid statistical foundation."
Study Details and Dataset
Presented at the 2024 American Society for Radiation Oncology (ASTRO) meeting, the study encompassed a significant dataset from 235 patients treated at the Miami Cancer Institute from 2017 to 2022, accounting for 1,503 brain metastasis cases across 358 SRS treatments. Advanced propensity score matching was utilized to mitigate confounding factors.
Patient Cohort Profile
The research comprised a diverse cohort, with a median age of 65 years and 61% being female. The patients had a median KPS of 90, and an average of four lesions treated during each SRS course. Notably, lung cancer predominated as the primary tumor type (58.5%), followed by breast cancer (24.6%). Radiation doses were administered as follows: 20 Gy for 20% of lesions, 22 Gy for 29%, and a substantial 51% received 24 Gy.
Machine Learning Insights
"We harnessed machine learning algorithms to identify the factors linked to local failure, allowing us to potentially foresee a patient's risk after radiosurgery," Kotecha explained.
Local Failure Statistics
With an average follow-up period of 12 months, local failure was recorded in 138 lesions, representing 9.2% of cases. Utilizing propensity score matching, 276 lesions from 123 patients were included in a generalized estimating equations model. Impressively, the machine learning model achieved an 88% overall accuracy and a remarkable 91% specificity at the one-year mark, along with an area under the curve of 0.8.
Implications for Follow-Up and Monitoring
Beyond enhancing dosage optimization, the AI tool has far-reaching applications in patient follow-up initiatives. By basing follow-up MRI scan frequencies on personalized failure risks, clinicians can reduce unnecessary imaging for low-risk individuals while intensifying monitoring for high-risk patients.
Future Prospects
"This initial model for predicting local failure tied to dosage offers clinical benefits in two ways," Kotecha noted. "As we enlarge our dataset by incorporating information from various institutions, we anticipate improving our predictive capabilities." Looking ahead, this model’s robustness may increase as more varied patient datasets from additional medical centers are integrated, allowing for a comprehensive exploration of its applicability in different clinical settings.
Conclusion
In today's evolving medical landscape, this pioneering AI tool might just reshape the treatment approach for patients battling brain metastases, potentially leading to better outcomes and personalized healthcare solutions.