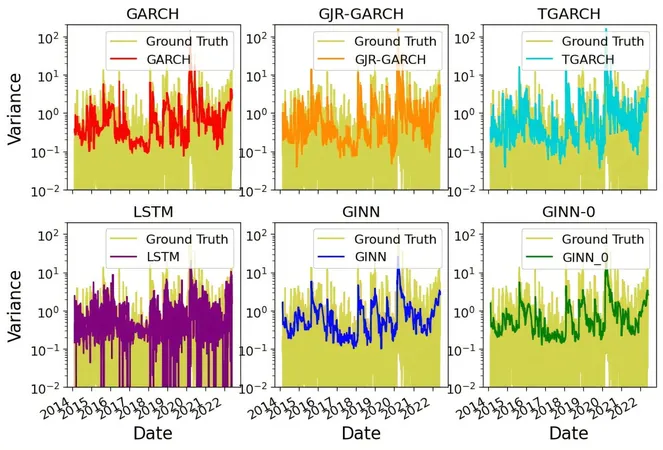
Revolutionary Hybrid Machine Learning Model Predicts Financial Market Volatility Like Never Before!
2024-12-17
Author: Nur
Introduction
Groundbreaking advancements in statistical modeling have always tantalized investors, especially in an era where volatility directly influences the risks and returns of their investments. This pursuit of enhanced prediction techniques once earned a Nobel Prize for methods that successfully captured time-varying volatility. Yet, many existing models, particularly variants of the autoregressive conditional heteroskedasticity (ARCH), fall short in effectively generalizing across diverse market conditions, largely due to their limitations in addressing nonlinear market behaviors.
The GARCH-Informed Neural Network (GINN)
Now, researchers at Carnegie Mellon University’s Department of Mechanical Engineering have unveiled a hybrid deep learning model that is set to change the game. This innovative approach combines the best of GARCH (Generalized Autoregressive Conditional Heteroskedasticity) with the dynamic capabilities of long short-term memory (LSTM) deep neural networks, resulting in a model that forecasts market volatility with superior accuracy compared to either method alone.
Drawing inspiration from physics-informed machine learning—a cutting-edge technique that integrates established physical laws within the framework of deep learning—this team has developed the GARCH-Informed Neural Network (GINN). This model cleverly intertwines the strengths of empirical observations captured by the GARCH framework with the predictive power of advanced machine learning, allowing it to recognize both overarching market trends and intricate fluctuations.
Challenges in Traditional Machine Learning
"We've encountered challenges with traditional machine learning techniques that often lead to 'overfitting,' where models replicate their training data too closely," explained Zeda Xu, a Ph.D. student at CMU and the lead author of the study presented at the prestigious ACM International Conference on AI in Finance. “Our hybrid approach emphasizes generalizability and boosts accuracy across varied scenarios."
Performance and Implications
The results speak for themselves—GINN outperformed the GARCH model by an impressive 5% when predicting the volatility of daily closing prices across seven major global stock market indexes. This innovation holds great promise not only for investors reliant on the GARCH model but also for an array of applications in time series modeling and forecasting, such as in autonomous vehicles and generative AI technologies.
Conclusion
"This represents a monumental leap for engineering, demonstrating how interdisciplinary techniques can yield transformative results across multiple domains," noted Chris McComb, an associate professor of mechanical engineering at CMU. “By merging insights from physics-informed machine learning with practical applications and expert collaboration, we are paving the way for robust time series forecasting models."
The implications are monumental: With GINN at their disposal, investors could navigate the unpredictable waters of the financial markets with greater confidence, potentially leading to more informed decisions and improved financial outcomes. As the financial landscape continues to evolve, this cutting-edge model is one to watch closely!
Stay tuned for more updates!
Stay tuned for more updates on innovations shaping the future of finance!