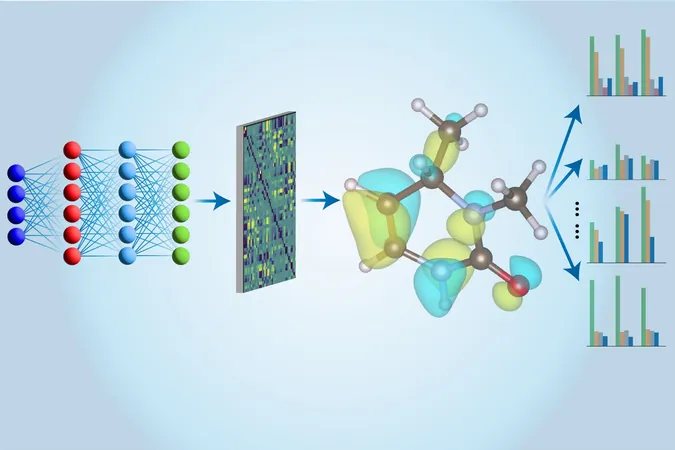
Revolutionary Machine Learning Techniques Propel Chemistry into the Future
2025-01-14
Author: Arjun
In a fascinating journey through history, our understanding of material design has evolved dramatically. Once upon a time, ambitious alchemists, including renowned figures like Tycho Brahe and Isaac Newton, devoted their lives to transmuting lead into gold. However, this dream was rooted in superstition rather than science. Fast forward to today, we possess advanced tools that make predicting and creating materials significantly more feasible.
The field of materials science has progressed by leaps and bounds thanks to the periodic table, which lays out the unique properties of elements, letting scientists know that, unlike ancient beliefs, elements cannot simply turn into one another. In recent years, advancements in machine learning have further propelled our capacity to analyze and predict the behaviors of molecules. A groundbreaking study led by Ju Li, a prominent MIT professor, promises to transform this arena by enhancing computational chemistry techniques, as detailed in the December 2024 issue of Nature Computational Science.
Traditionally, researchers have relied heavily on density functional theory (DFT) to calculate the properties of molecules, a method that has proven effective but comes with limitations. While DFT provides estimations on molecular systems' total energy based on electron density distribution, it's not uniformly accurate and offers limited insights.
The solution? Enter coupled-cluster theory (CCSD(T)), deemed the "gold standard" of quantum chemistry by Li. Despite its unmatched accuracy, CCSD(T) calculations are computationally intensive and traditionally constrained to smaller molecules due to the exponential increase in time and resources needed for larger systems.
However, the innovative team at MIT has ingeniously merged traditional CCSD(T) calculations with machine learning to expedite this process. By first conducting calculations on conventional computers, they then train a state-of-the-art neural network—dubbed the Multi-task Electronic Hamiltonian network (MEHnet)—to analyze various molecular properties at astounding speeds. This network not only predicts molecular energy but also characterizes multiple electronic properties in one go, leading to a more holistic understanding of molecules.
This intelligent system has already shown remarkable success in evaluating known hydrocarbon structures, often outperforming classical DFT methods and aligning closely with experimental results. Experts like Qiang Zhu of the University of North Carolina at Charlotte praise the project, highlighting its potential for effective analyses with smaller datasets while maximizing accuracy and efficiency.
As the MIT team has begun expanding their work beyond simple elements to include heavier elements like silicon and platinum, they are paving the way for future innovations. Li suggests that the capabilities of their model could eventually extend to analyzing large molecules containing thousands of atoms – a feat that was previously inconceivable.
The implications are already stirring excitement across various sectors. Researchers envision applying this advanced model to probe uncharted materials and design novel substances with specific properties tailored for technological advancements in drug development, solar cells, and battery creation.
One of the enticing prospects readers can hold on to is the potential for high-throughput molecular screening. As this approach matures, it promises to streamline the identification of new compounds, paving the way for innovative breakthroughs that could resonate throughout industries.
Looking into the future, Li expresses ambitious goals: achieving CCSD(T)-level accuracy for an entire range of elements in the periodic table but at a fraction of the computational expense associated with DFT. This game-changing research could unlock solutions to pressing challenges in chemistry, biology, and materials science we are yet to fully comprehend. The horizon ahead is vast and full of possibilities. What new materials could we discover? What transformative technologies could emerge from this exploration? The excitement is palpable, and the scientific community waits breathlessly for what comes next.