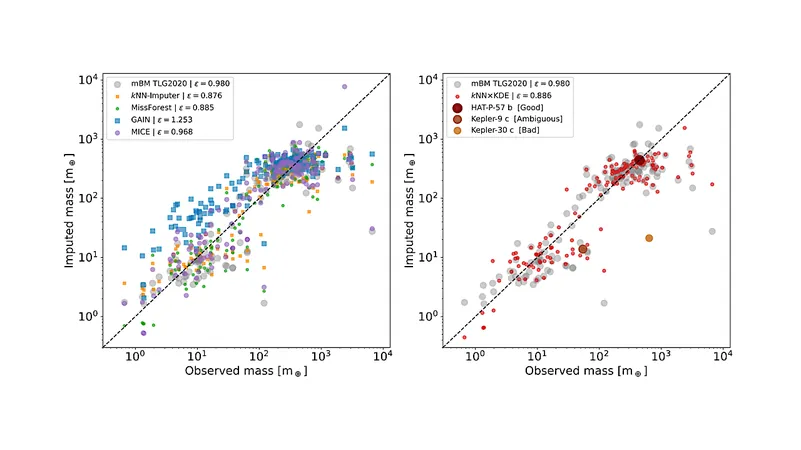
Revolutionary Machine Learning Techniques to Estimate Exoplanet Mass from Incomplete Data!
2024-10-11
Author: Siti
Introduction
In the vast realm of astrophysics, the exoplanet archive stands out as a treasure trove filled with vital information about discovered extrasolar planets. However, one pressing issue hampers optimal statistical analysis: a staggering number of missing values. Particularly, estimating the mass of these planets—one of the most critical characteristics—is a daunting task, as over 70% of known exoplanets lack a measured mass.
Objective
Recent research undertook the task of leveraging machine learning to address this challenge by comparing five different algorithms capable of utilizing incomplete datasets. The aim was to estimate the elusive planet mass by imputing the missing data. The study scrutinized outcomes using two distinct approaches: one that involved a partial dataset with six complete planet properties and another that harnessed a more comprehensive set of incomplete properties totaling six and eight.
Findings and Results
The findings are groundbreaking: the accuracy of mass predictions significantly improved with the availability of additional data, even when that data was only partially complete. This advancement paves the way for mass predictions for all documented planets, regardless of the properties that are known. The researchers honed in on an innovative algorithm named kNN×KDE, which not only predicts values but also yields a probability distribution for the imputed properties. This distribution shape is instrumental in assessing the algorithm's confidence levels and revealing insightful demographics regarding exoplanet populations.
Applications and Implications
To illustrate the capabilities of this algorithm, the researchers provided numerous examples involving planets discovered through both transit and radial velocity methods. Impressively, they also tested the generative power of kNN×KDE to create an extensive synthetic population of planets, uncovering distinct potential categories based on grouped properties in a multidimensional framework.
Conclusion
With all coding available as Open Source, this research opens new avenues in the study of exoplanets, providing not only tools for analysis but also exciting opportunities for further discoveries.
Publication and Future Directions
This groundbreaking paper, featuring 30 pages packed with intricate figures and detailed data, has already garnered attention and been accepted for publication in the prestigious Open Journal of Astrophysics. The implications of this work extend into Earth and planetary astrophysics, advanced instrumentation, and even machine learning fields, signaling a remarkable leap in our quest to uncover the mysteries of the universe. Stay tuned as astronomers and machine learning experts alike delve deeper into this fascinating research, potentially reshaping our understanding of exoplanet demographics and properties!