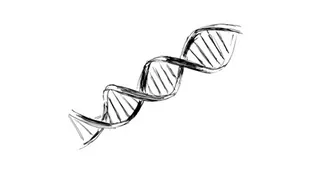
Revolutionary Machine Learning Tool Set to Transform Stereotactic Radiosurgery for Brain Metastases
2024-10-07
Author: Yu
In a groundbreaking development at the 2024 American Society for Radiation Oncology (ASTRO) Annual Meeting, Dr. Rupesh Kotecha introduced a pioneering machine learning tool designed to predict local failure after stereotactic radiosurgery (SRS) for patients with brain metastases. Utilizing a robust dataset of 1,503 treatments across 235 patients, the tool evaluates three different radiation doses: 20, 22, and 24 Gy.
Remarkably, the tool has demonstrated an impressive accuracy rate of 88%, with specificity hitting 91% and sensitivity at 89% over two years. These figures underscore its potential to not only determine optimal radiation dosages but also individualize MRI scan schedules according to each patient’s risk profile.
Dr. Kotecha, a radiation oncologist at Miami Cancer Institute, outlined that the need for such predictive tools stems from the inherent uncertainties in patient outcomes following treatment. Typically focused on small, intact brain metastases under 2 cm, the study seeks to uncover modifiable and non-modifiable factors that contribute to local failure after radiosurgery.
The dataset utilized for the machine learning model was meticulously curated, spanning patients treated from January 2017 to July 2022. The research employed advanced statistical methods, including propensity score matching analysis, to analyze a wealth of treatment-related data and patient characteristics, aiming to predict the likelihood of local failure over time.
Among the key findings is a new clinical decision-making tool that can tailor radiation dosages effectively. If certain doses do not significantly enhance treatment outcomes, they can be omitted, sparing patients from unnecessary exposure. Furthermore, the tool allows clinicians to optimize the timing of follow-up MRI scans based on individual risk assessments.
As Kotecha explained, developing the machine learning algorithm necessitated a well-curated dataset, emphasizing that the efficacy of the model relies heavily on the quality of the input data. With standardized treatment practices in place, the Miami Cancer Institute has been able to gather reliable data crucial for such innovations.
Looking ahead, the researchers plan to expand the model's applicability by incorporating systemic therapy effects and validating results with diverse patient populations. This includes adapting the model for various demographics, such as European populations, which may require adjustments.
Excitingly, in alignment with advancing technology, Dr. Kotecha mentioned the development of an app-based system that will allow healthcare providers to input key parameters such as patient age, gender, and tumor characteristics. In real-time, the system will calculate the risk of local failure at 6 months, 1 year, and 2 years, helping practitioners select the most effective radiation dose tailored to their patients.
In conclusion, this innovative tool represents a significant step forward in the realm of oncological treatments, offering the promise of personalized care that could revolutionize outcomes for patients battling brain metastases. As the study progresses towards publication, the broader implications for predictive analytics in cancer treatment are both profound and compelling. Stay tuned for more updates as this technology evolves!