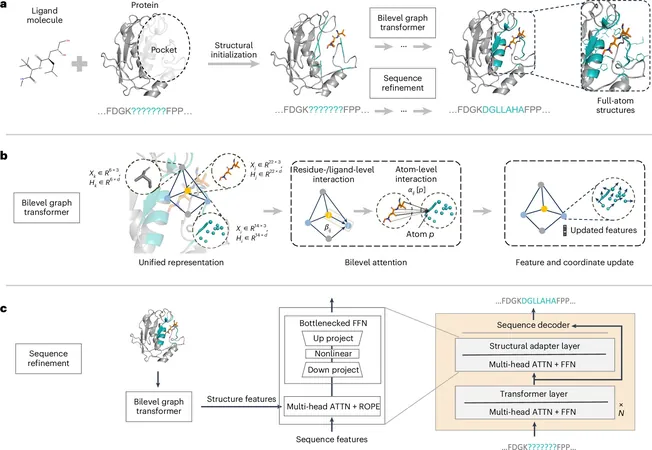
Revolutionary New Algorithm for Protein Design Leaves Traditional Methods in the Dust!
2024-12-09
Author: Wei Ling
Introduction
In a groundbreaking study published in *Nature Machine Intelligence*, researchers from the University of Science and Technology of China (USTC) have unveiled PocketGen, a cutting-edge deep generative algorithm that is set to transform the field of functional protein design. Led by the brilliant Prof. Liu Qi and in collaboration with the Marinka Zitnik lab at Harvard Medical School, this innovative approach marks a significant leap forward, eclipsing conventional methods that have long been deemed tedious and inefficient.
Importance of Functional Protein Design
Functional protein design is essential for developing proteins that bind to small molecules, playing a pivotal role in drug discovery and various biomedical applications. Historically, methods reliant on energy optimization and template matching not only consumed considerable time but also achieved disappointing success rates.
Introduction of PocketGen
Enter PocketGen, a hero in the realm of protein modeling, designed to overcome the inherent challenges that face traditional deep learning models, such as accurately representing complex molecular interactions and understanding sequence-structure dependencies.
Key Components of PocketGen
So, what makes PocketGen stand out? This novel algorithm is woven from two key components that capitalize on cutting-edge advancements in molecular modeling. First, it incorporates a sophisticated dual-layer graph Transformer encoder, inspired by the intricate hierarchical structures of proteins. This feature adeptly captures various fine-grained interaction details, updating amino acid representations and spatial coordinates with precision.
The second game-changing element of PocketGen is a pre-trained protein language model. This model effectively fine-tunes the ESM2 framework to enhance the prediction of amino acid sequences. By selectively adjusting parameters, PocketGen ensures a superior consistency between sequence and structure, leveraging advanced cross-attention mechanisms that increase its predictive power.
Performance and Validation
What are the results? PocketGen not only promises but delivers astonishing performance, having achieved over a tenfold increase in speed compared to traditional frameworks. It has been validated through rigorous comparison with leading generative models, including those developed by the esteemed Nobel Laureate David Baker's lab. The algorithm demonstrated remarkable effectiveness in protein pocket design tasks for small molecules like fentanyl and ibuprofen.
Interpretability and Future Implications
Moreover, the interpretability of PocketGen adds another layer of appeal. By comparing its attention matrices with results derived from first-principle-based force field simulations, researchers were able to verify that PocketGen doesn’t just work remarkably well—it also makes sense scientifically.
Conclusion
This development could be a game-changer for researchers and pharmaceutical companies alike, streamlining the pathway to innovative drug designs and advanced medical research. As the pace of scientific inquiry accelerates, PocketGen is poised to be a vital tool in the fight against complex diseases. Keep an eye on this revolutionary technological advancement—it's only just beginning to demonstrate its true potential!