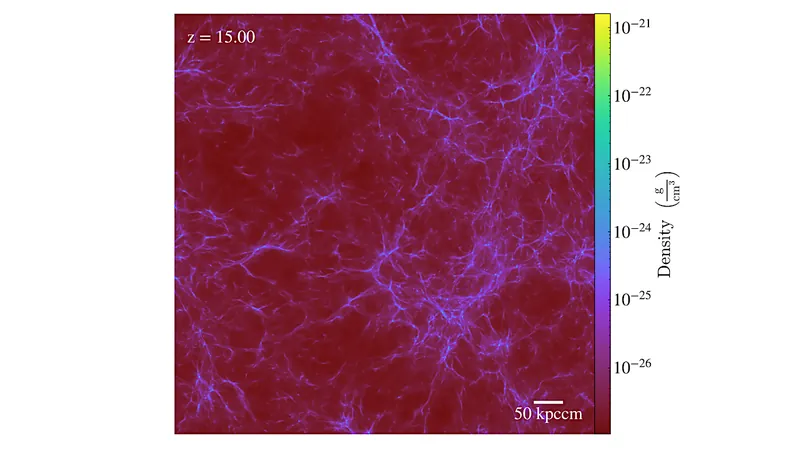
Revolutionizing Astrophysics: How Neural Operators Are Transforming Chemical Evolution Simulations!
2025-03-17
Author: John Tan
Introduction
In a groundbreaking study, researchers are harnessing the power of machine learning, particularly through the use of Neural Operators, to enhance the Grackle chemistry solver—an essential component in cosmological hydrodynamical simulations. This innovative approach promises to redefine how we understand chemical evolution in the cosmos!
Neural Operators and Their Role
Neural Operators allow for a mesh-free and data-driven methodology to solve complex, coupled ordinary differential equations that dictate chemical evolution, as well as gas cooling and heating processes. The researchers constructed and trained multiple variants of DeepONet—an advanced architecture of Neural Operators—using expansive datasets derived from current cosmological simulations.
Results
The results are impressive, with these machine learning models consistently reproducing Grackle's outputs and achieving an average error of less than 0.6 dex under typical conditions. However, the accuracy tends to falter in ultra-dynamic chemical environments, revealing areas for further exploration.
Computational Speed Improvements
Notably, these machine learning models provide significant computational speed improvements—up to six times faster than traditional methods—streamlining large-scale simulations and potentially alleviating long-standing computational bottlenecks in astrophysical modeling.
Challenges Ahead
Nonetheless, some hurdles remain. Specifically, iterative applications face challenges due to error accumulation, which can lead to numerical instability. Moreover, the efficacy of these models is closely tied to the quality of the training datasets utilized and struggles with the extrapolation abilities characteristic of deep learning technologies.
Future Directions
While these Neural Operator-based emulators show immense potential, they require further refinement for seamless integration into astrophysical simulations. Looking ahead, the researchers emphasize the need for improvements in stability over iterative time steps and optimization for hardware acceleration.
Conclusion
This study is merely the first step on the path toward the widespread incorporation of machine learning in astrophysical chemistry solvers, paving the way for exciting advancements in our understanding of the universe! With ongoing developments in this field, the future of astrophysics looks brighter than ever. Don’t miss the chance to see how these cutting-edge technologies could unlock further mysteries of the cosmos!