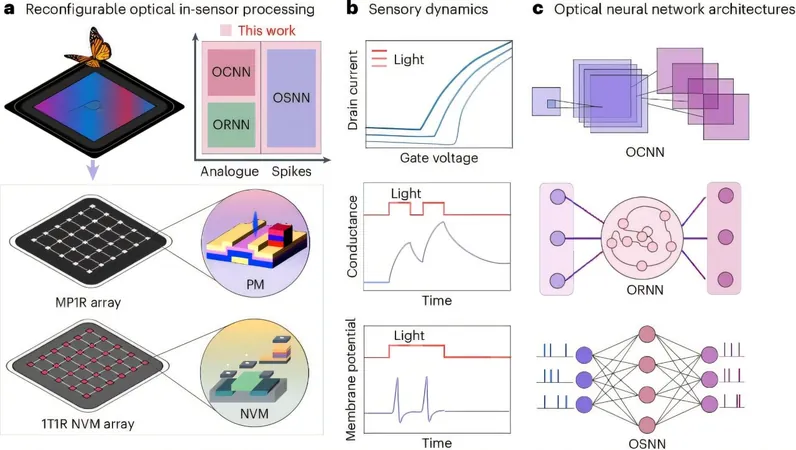
Revolutionizing Computing: A New Device Merges Sensing and Processing, Taking AI to the Next Level!
2024-12-08
Author: Ming
Introduction to Revolutionary Computing Device
In a groundbreaking development that could reshape the landscape of artificial intelligence, engineers at Peking University have successfully devised a revolutionary reconfigurable computing platform that integrates both sensing and processing functions into a single device. This innovative system, detailed in a recent publication in Nature Electronics, utilizes an intricate array of phototransistors combined with Mott memristors, dubbed the MP1R, to tackle the mounting computational demands of machine learning algorithms.
Challenges with Traditional Computing Systems
“Traditional computing systems, particularly those relying on CMOS von Neumann architecture, face severe limitations, particularly in real-time image processing,” stated Yuchao Yang, the senior author of the study, in an interview with Tech Xplore. He emphasized that the physical separation between sensors, memory, and processors in typical systems leads to inefficiencies that manifest as increased power consumption and processing latency. In contrast, biologically inspired vision systems, like the human eye, offer an elegant solution due to their remarkable efficiency and adaptability, motivating their research team's work toward creating an architecture inspired by natural processes.
Creating a Universal In-Sensor Processing Platform
Despite the promise shown by photonic memristors in computer vision tasks, their capabilities have often fallen short when it comes to processing optical data for diverse neural network architectures. In response to this limitation, the Peking University team sought to create a universal in-sensor processing platform that could seamlessly accommodate varied computational needs beyond mere computer vision applications.
Innovative Design Features
“By integrating a 20x20 array of phototransistors with 20 reconfigurable Mott memristors, we aimed to enhance the platform's flexibility significantly,” Yang elaborated. The innovative approach began with the fabrication of amorphous indium gallium zinc oxide (α-IGZO) thin-film transistors, enabling sophisticated back-gate phototransistors capable of detecting light across a spectrum of wavelengths.
This new array not only detects light but also adjusts its reactive processes according to specific wavelengths—exhibiting potentiation behavior under blue light and depression when exposed to red. The integrated Mott memristors, constructed from Ta/TaOx/NbOx/W heterostructures, add crucial functionalities, including a linear resistive region, volatile memory, and threshold switching, allowing the system to effectively simulate synaptic and neuronal behavior.
Compatibility with Neural Network Architectures
One standout feature of Yang’s platform is its compatibility with various neural network architectures, such as Convolutional Neural Networks (CNNs), Recurrent Neural Networks (RNNs), and Spiking Neural Networks (SNNs)—signaling vast potential applications in real-world scenarios. “Our approach not only bridges the gap between biologically inspired and machine-learning algorithms but also facilitates simplified circuit design by consolidating diverse neural processing principles into one compact device,” Yang explained.
Benefits of the New Architecture
This unification results in a streamlining of network complexity, yielding benefits such as lower latency and improved energy efficiency—a critical asset for real-time image processing applications. Moreover, the platform shows remarkable reliability across multiple tasks, thanks to its lower variability in the Ta/TaOx/NbOx/W memristors.
Future Implications and Developments
The implications of this research are profound, potentially laying the groundwork for large-scale, energy-efficient, and low-latency neuromorphic vision systems. “As we continue to advance this technology, we envision it playing a vital role in pushing the boundaries of advanced AI applications, promoting flexible, high-performance solutions,” Yang stated.
Looking Ahead
Looking ahead, the research team aims to focus on enhancing the device's integration density and computational efficiency through three-dimensional structures. Moreover, improvements in power consumption and sensitivity to lighting will further enhance the platform's versatility, enabling effective data collection in various environmental conditions.
Conclusion: A Leap in AI Technology
In summary, this pioneering study not only marks a significant step forward in neuromorphic vision systems but also opens the door to future innovations in artificial intelligence by combining the best features of biological vision with cutting-edge computational capabilities. As the demand for more powerful and adaptable AI technology continues to grow, this development could very well be a game changer, heralding a new era of intelligent systems. Stay tuned for future breakthroughs in this exciting field!