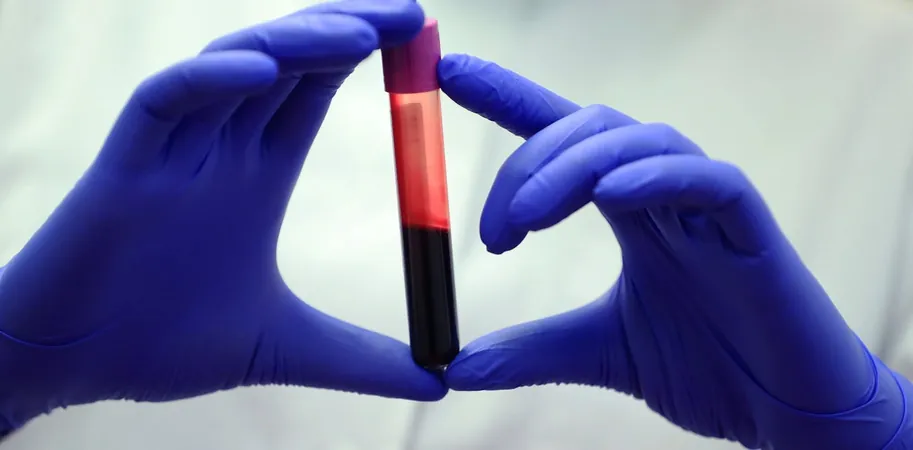
Revolutionizing Health: How Machine Learning Can Shrink ‘Normal’ to Fit You!
2024-12-11
Author: Wei Ling
Have you ever undergone a basic blood test? If so, you're likely familiar with the complete blood count (CBC)—one of the most prevalent blood tests performed across the globe. Billions of these tests are conducted annually, serving vital roles in diagnosing health conditions and monitoring ongoing health changes.
However, despite its widespread use, the interpretation of CBC results often lacks the precision needed for effective patient care. Currently, medical professionals employ standardized reference intervals that often overlook individual variations, presenting significant limitations in patient diagnosis and treatment.
Recently, a team of researchers, including mathematicians from the University of Washington School of Medicine, has set out to change that. They have developed innovative computational methods aimed at redefining what constitutes “normal” blood counts for each patient, enabling more accurate assessments of health risks.
Decoding the Clinical Test Landscape
Most people view clinical tests simply as tools for diagnosis. For instance, results from tests such as COVID-19 or pregnancy tests are clear-cut—positive or negative. Yet, blood tests like the CBC measure biological components that fluctuate within certain ranges rather than providing dichotomous outcomes.
A CBC test unveils a comprehensive profile of your blood components, including red blood cells, platelets, and white blood cells. For instance, hemoglobin levels indicate oxygen transportation efficiency in the blood, while platelet counts reveal bleeding tendencies, and white blood cell counts signal an immune response to infections.
The Challenge of Reference Intervals
This situation begs the question: What exactly defines high or low results in blood tests? Typically, clinicians determine reference intervals by analyzing blood samples from healthy individuals and establishing the middle 95% of values. However, this traditional approach fails to consider the unique biological makeup of each patient.
Many blood markers are influenced by genetic and environmental factors, meaning what is "normal" for one person can be distinctly different from what’s "normal" for another. For example, while the general platelet count is defined as 150 to 400 billion cells per liter, you may have a personal set point around 200, drastically narrowing your true normal range.
Such discrepancies can critically impact diagnoses. If a patient's set point diverges significantly from standard cutoffs, doctors may overlook potential diseases—or conversely, conduct unnecessary tests if a patient’s values hover close to a cutoff.
Personalized Medicine is Within Reach
Thankfully, routine blood testing provides a pool of data to work from. By leveraging machine learning techniques, the research team analyzed historical blood count data from over 50,000 patients, unveiling personal blood count set points derived from patients’ clinical histories. They found that individual normal ranges are generally three times smaller than population averages.
For example, while the widely accepted normal range for white blood cell counts hovers between 4.0 to 11.0 billion cells per liter, the true individual ranges often cluster between 4.5 to 7, and sometimes even tighter, between 7.5 to 10. This more personalized approach represented a paradigm shift in diagnosing conditions like iron deficiency, chronic kidney disease, and hypothyroidism, drawing attention to anomalies that might otherwise evade detection.
Intriguingly, the researchers also discovered that these individual set points are strong indicators of future health risks. Patients with elevated white blood cell set points were more susceptible to developing Type 2 diabetes, exhibiting nearly double the mortality risk compared to those with lower counts. Other markers were similarly telling, suggesting robust predictive power when it comes to future health.
The Future is Here: Personalized Health Monitoring!
Imagine a medical landscape where your own historical data shapes your health assessments—a world increasingly democratized by personalization in healthcare. As we venture into this exciting frontier, healthcare providers may soon adopt these individualized set points to sharpen disease screenings and enhance diagnostic interpretations.
With ongoing advancements powered by machine learning, what was once an impersonal and generalized approach to health is transforming into a bespoke medical experience. Get ready for a future of healthcare uniquely tailored just for you!