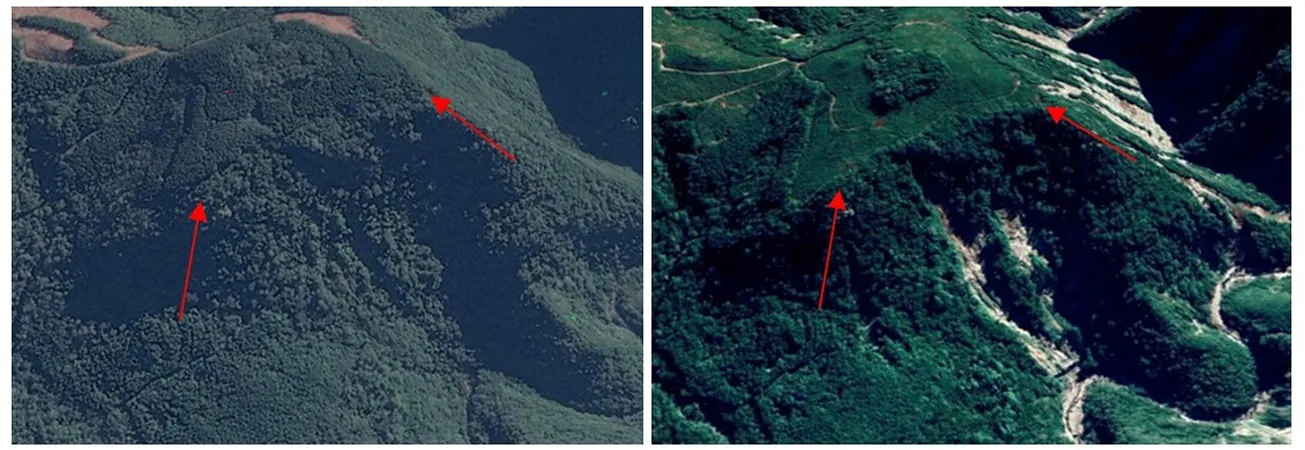
Revolutionizing Landslide Analysis: New Algorithm Slashes Classification Errors!
2024-12-20
Author: Arjun
Introduction
In the realm of environmental science, analyzing land use and land cover (LULC) has become a cornerstone for understanding ecological changes and climatic trends. This analysis not only provides insights into the natural world but also plays a pivotal role in assessing the risks of natural disasters such as landslides. As changes in LULC can drastically alter the ecological balance, they often heighten the vulnerability of certain terrains, especially in mountainous regions known for their susceptibility to hazards.
The Challenge of Classification Errors
One of the foremost challenges in LULC analysis is the presence of classification errors, which can distort the understanding of environmental transitions. These errors can lead to "invalid transitions," or changes in land cover that are unlikely to have occurred. Such misinterpretations can obscure the real causes and impacts of hazardous events, potentially exacerbating disaster risks.
Introducing RF-CMAP: A New Methodology
In a groundbreaking study, a collaborative team of researchers from Brazil, Ecuador, and China has introduced an innovative method that combines the power of the Random Forest (RF) algorithm with the temporal perspective of the Compound Maximum a Posteriori (CMAP) algorithm, aptly named RF-CMAP. This new methodology streamlines the classification process by considering the temporal dynamics of land cover changes, resulting in a more accurate reflection of natural processes.
A Closer Look at RF-CMAP: Accuracy Beyond Expectations!
By leveraging years of data from Landsat images (specifically from 2000, 2008, and 2016), the RF-CMAP model significantly outperformed traditional methods. While both the RF and RF-CMAP models yielded impressive overall accuracy rates above 0.87, the RF-CMAP methodology excelled across all analyzed years, rectifying an impressive 99.92 km² (12%) worth of invalid transitions noted in the RF classifications.
The benefits of the RF-CMAP approach were clearly demonstrated in validation analyses, where it achieved an accuracy rate of 0.703 for LULC change areas between 2000 and 2008—a considerable jump from the 0.622 accuracy rate of the RF model. The error correction rate for RF-CMAP further increased to 81% during the subsequent period of 2008 to 2016.
Additionally, RF-CMAP made significant strides in mitigating the "salt-and-pepper" effect that often plagues classified images, which enhances the coherence of classified regions. It also excelled in accurately identifying unchanging land covers such as forests, bare soil, and water, correcting 50% more errors than the RF model during the earlier timeframe.
A Response to Natural Disasters: Targeting Landslide Risks
The study focused on the Rolante River Basin, which faced catastrophic landslides in 2017 after heavy rainfall triggered over 300 incidents. This extreme weather combined with LULC changes raises the stakes for soil stability, making accurate LULC change detection critical for mitigating future disasters.
Notably, the research uncovered that 35% of the landslides were linked to invalid transitions recorded between 2000 and 2008, with 16% tied to transitions from 2008 to 2016. For instance, when misclassifications suggested reforestation had occurred, it led to incorrect judgments about soil conditions. The RF-CMAP method proved its mettle by accurately classifying 66% of the landslide-affected zones versus a mere 21% with traditional RF classification.
The Future of Disaster Risk Management is Here!
This innovative research underscores the immense potential of combining advanced technologies like RF and CMAP to enhance the accuracy of LULC change analysis. By addressing invalid transitions specifically in landslide-prone areas, this sophisticated model not only aids in disaster risk management but also helps protect vulnerable communities from future catastrophes.
As remote sensing technologies and predictive analytics advance, their integration into sustainable management and disaster preparedness strategies will become essential. Researchers believe the widespread adoption of methodologies like RF-CMAP could herald a new era of safety and sustainability in the face of natural adversities. So, get ready—this revolution in environmental science could redefine how we understand and interact with our planet!