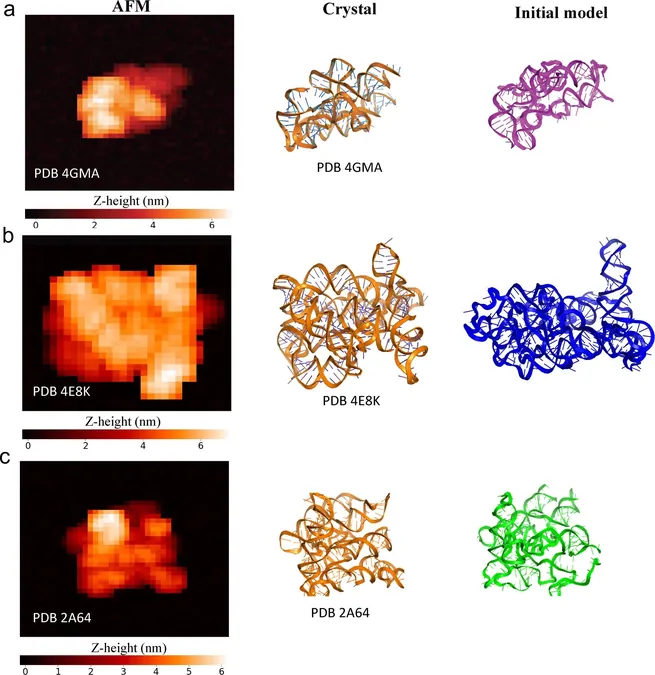
Revolutionizing RNA Research: Meet HORNET, the Game-Changing Visualization Technique!
2024-12-31
Author: Mei
Introduction
Researchers at the National Cancer Institute have unveiled a groundbreaking method called HORNET, designed to explore the intricate 3D topological structures of large and flexible RNA molecules. This innovative approach leverages atomic force microscopy (AFM) in conjunction with deep neural networks and unsupervised machine learning, enabling scientists to observe individual RNA conformers under physiological conditions.
The Challenges of RNA Complexity
The complexity of human RNAs arises from various structural elements that play pivotal roles in biological functions. Traditional methods, like cryo-electron microscopy, often rely on samples that are not only homogeneous but also average signals to discern structures. Large and flexible RNAs remain particularly elusive due to their tendency to adopt multiple conformations when in solution, making them challenging to analyze effectively.
The Gap in Structural Biology
Until now, no comprehensive database has linked RNA sequences to their corresponding 3D structures, creating a significant gap in the field of structural biology. Unlike successful protein-focused methods, such as the well-known AlphaFold, RNA-specific techniques have not been thoroughly developed, reflecting the difficulties in capturing consistent structural models for these vital molecules.
Study Overview
In a recent study published in *Nature*, titled "Determining structures of RNA conformers using AFM and deep neural networks," researchers provide an in-depth exploration of HORNET's capabilities to unveil previously hidden structural features of large and flexible RNAs.
Methodology
To achieve their findings, researchers collected single-molecule AFM images of benchmark RNAs in various conformations. They then utilized unsupervised machine learning and advanced deep neural networks to correlate molecular topographies with energy distributions. The training of their system incorporated a pseudo-structure database that encompassed a wide range of RNA folds, and it was rigorously tested on RNAs exceeding 200 nucleotides, including RNase P RNA, cobalamin riboswitches, group II introns, and the HIV-1 Rev response element RNA.
Results and Accuracy
Results from the tests showed that HORNET could accurately reconstruct individual RNA conformations, often achieving root-mean-square deviations below the 7 Å threshold—a benchmark used in structural biology to confirm significant feature alignment in large RNAs. Additionally, benchmark experiments using both simulated and actual AFM images validated the technique's reliability in integrating established constraints and AFM pseudo-potentials.
Visualization of Conformations
The study revealed that different conformations of RNase P RNA and HIV-1 Rev response element RNA could be visualized at the single-molecule level. The estimated accuracies from the deep neural networks closely matched distances observed in known structures, underscoring HORNET’s precision.
Implications of HORNET
HORNET marks a significant advancement in RNA structural biology, offering a new direct method to explore challenging RNA structures. Its implications extend far beyond basic research, potentially impacting fields such as clinical diagnostics, pharmaceutical development, and biotechnology innovation.
Conclusion
As scientists continue to unravel the mysteries of RNA, HORNET stands at the forefront, promising to revolutionize our understanding of one of life's most essential molecules. Stay tuned for more updates, because this is just the beginning of RNA's exciting journey!