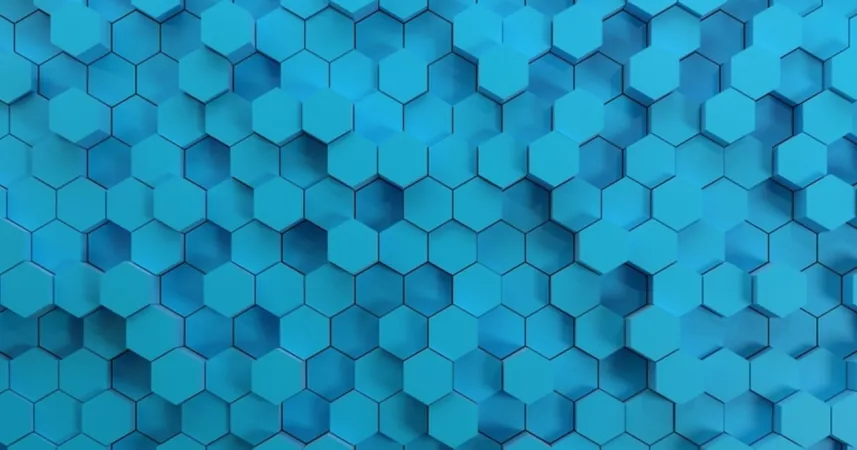
Transform Your AI Operations: Unlock Cost Savings with Google Cloud's Latest Insights
2025-04-09
Author: Mei
Google Cloud Unveils Game-Changing AI Optimization Tools
Google Cloud has launched an impressive array of new tools and features aimed at helping organizations cut costs and enhance the efficiency of their AI workloads. This initiative comes at a time when businesses are urgently searching for ways to streamline their AI expenditures while still delivering top-tier performance and scalability.
Focus Areas for AI Enhancement
The new features zero in on three essential aspects: optimizing compute resources, utilizing specialized hardware acceleration, and intelligent workload scheduling. These advancements tackle the critical challenge faced by enterprises—striking a balance between innovative AI deployment and effective cost management.
Direct Words from Google Cloud's VP of AI Products
According to Google Cloud's VP of AI Products, the new features are designed to help organizations address the crucial need to optimize AI-related costs without compromising on performance. These innovations provide more efficient strategies for running machine learning training and inference.
Smart Platform Choices for AI Deployment
Google Cloud encourages organizations to select the right platform wisely. From fully-managed services to highly customizable solutions, options abound. Vertex AI presents itself as a unified, fully-managed development platform, while Cloud Run supports scalable inference services with GPU capabilities. For extensive tasks, combining Cloud Batch with Spot Instances can drastically minimize costs. Businesses already using Kubernetes can leverage Google Kubernetes Engine (GKE), while those needing more control can opt for Google Compute Engine.
Maximizing Container Performance
A major tip for optimizing performance involves keeping containers lightweight. Google suggests using external storage options like Cloud Storage via FUSE, Filestore, or shared read-only persistent disks for inference containers in GKE or Cloud Run. This strategy not only cuts down on container startup times but also boosts scaling efficiency—key elements for managing performance and costs.
Selecting the Right Storage Solutions
Choosing the right storage becomes crucial in optimization. Google Cloud recommends Filestore for small AI workloads, while Cloud Storage caters to object storage needs at any scale. For high-speed requirements, Parallelstore offers sub-millisecond access times, and Hyperdisk ML is tailored for high-performance serving tasks.
Streamlining Resource Acquisition
To prevent expensive delays in acquiring resources, Google Cloud underscores the importance of tools like Dynamic Workload Scheduler and Future Reservations, which ensure that necessary cloud resources are secured ahead of time, enabling smooth operations.
Efficiency Through Custom Disk Images
A final strategy for enhancing deployment efficiency involves creating custom disk images. Instead of setting up operating systems, GPU drivers, and AI frameworks repeatedly, organizations can maintain custom disk images, allowing for the rapid deployment of fully-equipped workers in mere seconds.
A Competitive Landscape in AI Cost Management
As AI cost management becomes ever more vital across industries, competitors like AWS and Microsoft Azure are stepping up their game. AWS has rolled out cost-conscious tools within its SageMaker platform, including Managed Spot Training and model monitoring features for budget-optimizing performance. Likewise, Azure enhances its AI offerings through Azure Machine Learning, providing intelligent autoscaling and reserved capacity pricing.
In this evolving landscape, all three cloud giants—Google, AWS, and Azure—are ramping up their focus on hybrid flexibility, storage optimization, and GPU acceleration, empowering enterprises to make smarter scaling and funding choices.