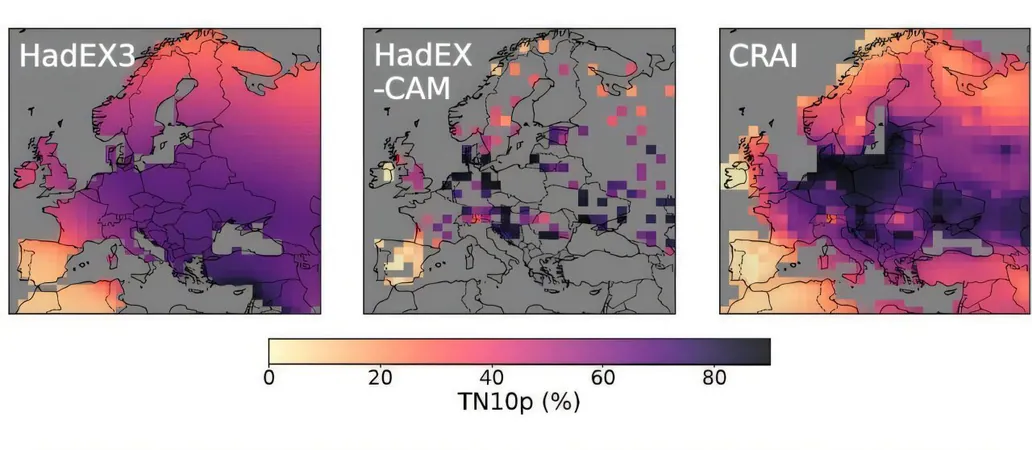
Uncovering Hidden Climate Extremes: How AI is Revolutionizing Historical Climate Analysis
2024-11-28
Author: Mei
Introduction
In a groundbreaking study, researchers have harnessed the power of artificial intelligence (AI) to analyze a treasure trove of climate data collected from over 30,000 weather stations worldwide, which measure crucial indicators like temperature and precipitation daily. Their revolutionary findings, published in *Nature Communications*, unveil previously unknown historical climate extremes, particularly in Europe—a region known for its dense network of weather stations with some records dating back to 1659.
Significance of the Study
As our planet's climate changes at an alarming rate, understanding how temperature and precipitation extremes are evolving is essential for resource planning and disaster preparedness. Recent studies indicate that rainfall is becoming heavier in various regions, pushing boundaries far beyond established climate norms. Similarly, extreme heat events have surged from 1% of global land areas experiencing monthly high temperatures above the two-sigma statistical level in 1950, to more than 30% today.
Challenge of Historical Data
One of the major challenges faced by climate researchers is the historical lack of comprehensive data, particularly from the early 20th century. There were times when weather stations remained unmonitored for extended periods due to various reasons, such as equipment failure or staff changes. Additionally, vast regions, especially in Africa and polar areas, are often poorly represented in historical climate records. Researchers typically use a process called data homogenization to address these gaps, but this method can introduce slight discrepancies in results among different studies.
AI Transformation in Climate Analysis
Led by Étienne Plésiat from the German Climate Computing Center in Hamburg, a team of researchers recognized an opportunity for applying AI’s sophisticated neural networks to the task of reconstructing past temperature extremes in Europe. Unlike traditional statistical methods, which often rely heavily on nearby station data, AI models excel when historical records are sparse or absent.
Results of AI Implementation
Utilizing advanced AI techniques, the team reconstructed records of extremely warm and cold days and nights across Europe. They compared their model's predictions against established climate simulations from the Coupled Model Intercomparison Project (CMIP6) and found that their AI approach, dubbed Climate Reconstruction AI (CRAI), significantly outperformed traditional interpolation methods. The accuracy of the CRAI technique was particularly notable when analyzing warm days, cool days, warm nights, and cool nights.
Assessment of Climate Extremes
The CRAI model was subsequently employed to assess the HadEX3 dataset, which encompasses more than 80 indices of extreme climate conditions from 1901 to 2018. This analysis not only reconstructed historical extremes, revealing spatial trends across critical time intervals but also identified previously unrecognized climate events, such as severe cold spells in 1929 and significant heat waves in 1911.
Future Implications
The researchers believe that this innovative approach holds tremendous potential for enhancing our understanding of climate extremes on a global scale, especially in regions plagued by data scarcity. They posit that further training of CRAI models, with access to larger datasets, could improve prediction accuracy even more.
Conclusion
This transformative research underscores the potential of AI to fundamentally enhance our grasp of climate change and its associated risks over time. With climate extremes being a growing concern worldwide, the implications of this study could be vital for future climate science and policy-making.
Further Reading
For those intrigued by the intersection of technology and environmental science, this study is a must-read, highlighting how AI can illuminate dark corners of historical climate data that have long been obscured.