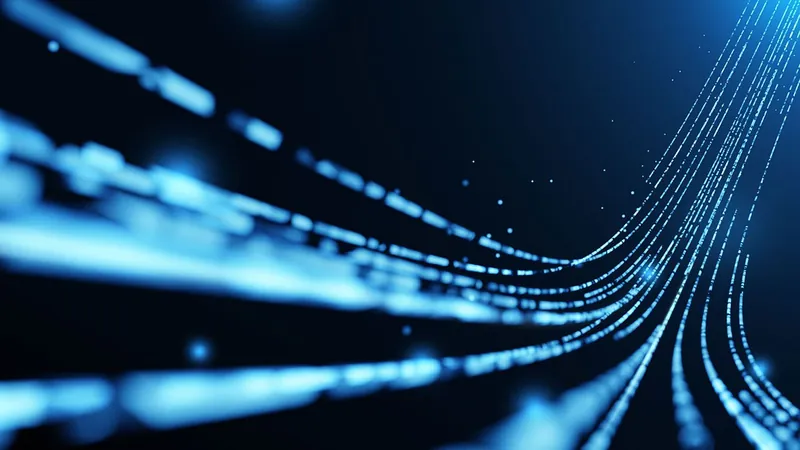
Unlocking the Power of AI: The Game-Changing Compression Technique for Local Use on Phones and Laptops!
2024-11-18
Author: Jia
Introduction
Large language models (LLMs) have revolutionized various domains, streamlining tasks from translation to customer service. However, leveraging their capabilities usually necessitates submitting requests to a centralized server, a method that is not only costly and energy-draining but can also be slow and cumbersome for users.
Groundbreaking Solution by Princeton and Stanford
Enter a groundbreaking solution developed by an innovative team of engineers from Princeton and Stanford universities. They have devised a cutting-edge algorithm designed to compress LLMs, paving the way for efficient local usage on devices like smartphones and laptops. This breakthrough could significantly enhance user privacy while simultaneously reducing energy consumption and associated costs.
Introducing CALDERA
This new approach, aptly named CALDERA (Calibration Aware Low precision DEcomposition with low Rank Adaptation), operates by eliminating redundancies and decreasing precision across an LLM's layers. The outcome? A more streamlined LLM that can be stored and accessed directly on personal devices while still delivering performance that rivals full-sized, uncompressed models.
Expert Insights
"As we reduce the demand for compute, storage, and bandwidth when using AI models, we open up accessibility for devices that otherwise couldn't handle such complex tasks," explained Andrea Goldsmith, the co-author and dean of Princeton's School of Engineering.
Rajarshi Saha, a Ph.D. student at Stanford and co-author of the study, highlighted that conventional models like ChatGPT involve expensive back-end processing. "By leveraging consumer GPUs for LLM inference, our goal is to facilitate this process with our compression techniques," he noted.
Upcoming Presentation at NeurIPS
The research team plans to unveil their findings at the prestigious Conference on Neural Information Processing Systems (NeurIPS) this December, where they will showcase the transformative potential of CALDERA. Their initial focus with this innovative strategy was on large datasets used to train AI models, which eventually evolved to include actual LLM compression.
Unique Integration of Techniques
What's particularly noteworthy about their algorithm is its unique integration of low-precision and low-rank techniques. While "low-precision" signifies a reduction in bits needed for storage, promoting faster processing and greater energy efficiency, "low-rank" focuses on minimizing redundancies within the weight matrices that LLMs utilize. By marrying these two concepts, the researchers achieved unprecedented levels of compression.
Testing and Results
Testing their method on Meta AI's open-source Llama 2 and Llama 3 models, Saha and his team discovered that this combined approach yielded performance improvements of up to 5% for critical tasks, notably in uncertainty metrics when predicting word sequences.
Benchmark Efficacy
To measure the efficacy of their compressed models, the team employed various benchmark tasks such as evaluating the logical order of statements and solving physical reasoning questions. Their results were promising, delivering strong performance and surprising accuracy within this new compression framework.
Broad Implications
The implications of this technology are vast. Compressed LLMs could function well in contexts where ultra-high precision isn't essential, making them an attractive option for individuals and businesses. Moreover, the ability to fine-tune these models directly on personal devices enhances privacy, mitigating the risk of data breaches when utilizing sensitive information.
Caution for Users
However, Saha warned potential users about the possible memory overload on devices when running LLMs, cautioning that excessive use could drain battery life. "We want to avoid scenarios where your phone runs out of charge in just an hour," he added. Low-precision computation can help address some energy concerns, but Saha stressed that this is just one part of a multifaceted solution.
Conclusion and Future Prospects
As the team continues to refine their algorithm, we may soon witness a significant shift in the accessibility and functionality of AI as it transitions seamlessly onto everyday personal devices. The future of mobile AI is on the brink of a breakthrough, and we're here for it!