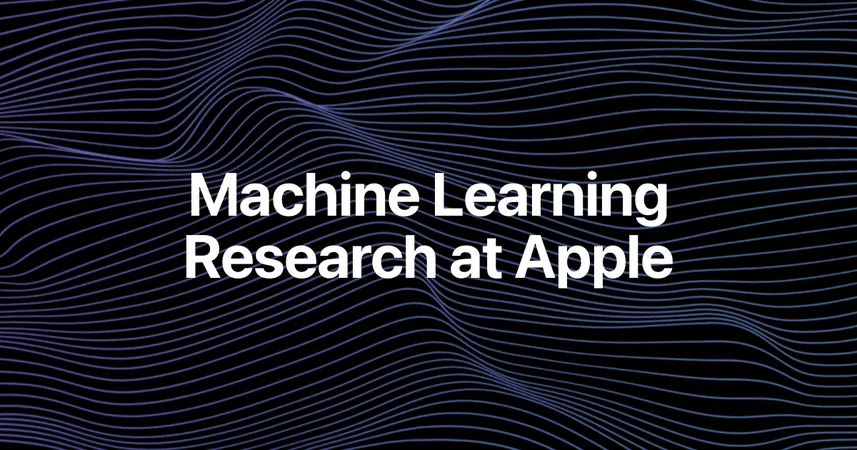
Unpacking Apple's Pioneering Workshop on Natural Language Understanding 2024
2025-04-07
Author: Li
Title: Unpacking Apple's Pioneering Workshop on Natural Language Understanding 2024
Apple's recent workshop on Natural Language Understanding (NLU) 2024 has shed light on the rapidly evolving technologies that enable more intuitive interactions with devices. With applications in prominent services like Siri and advanced search algorithms, NLU continues to reshape the way users engage with technology. The workshop convened leading experts from Apple and the academic community to explore the latest breakthroughs in large language models (LLMs).
The Dawn of Efficient Models
Recent years have witnessed LLMs becoming essential tools for tackling diverse tasks. Consequently, there is an increasing demand for more efficient modeling techniques. Notable presentations at the workshop emphasized innovative architectures as alternatives to the traditional attention-based transformer models.
Cornell University's Sasha Rush introduced State-Space Models (SSMs), which offer competitive accuracy and scalability. These models open the door to novel design possibilities, such as creating byte-level LLMs and enhancing inference speeds through model distillation. Meanwhile, Yoon Kim from MIT showcased advancements in Recurrent Neural Networks (RNNs) that utilize matrix-valued hidden states to improve training efficiency and processing throughput.
Yejin Choi from Stanford and NVIDIA further contributed by advocating for specialized distilled models tailored for specific applications, arguing that quality is paramount even over scale. This aligns with the sentiments of other researchers emphasizing the importance of targeted LLM applications.
Apple's Mehrdad Farajtabar shared insights into optimizing LLM inference on low-memory devices. Utilizing techniques like sparsity awareness and context-sensitive loading, Farajtabar's methods demonstrated substantial speedups—up to 25 times on GPUs.
Revolutionizing Reasoning and Planning
As LLMs expand their capabilities, improving their problem-solving and planning skills becomes crucial. The workshop illuminated the potential for LLMs to tackle complex requests by breaking them into manageable components using techniques like Chain of Thought and self-reflection.
Navdeep Jaitly from Apple shared experimental results demonstrating how hybrid approaches could enable smaller LLMs to generate strategies while larger ones execute them. This could maintain performance levels while enhancing planning capabilities. Moreover, UC Santa Barbara’s William Wang discussed how the identification of reasoning paths in training data could boost LLM performance by aggregating these connections.
Additionally, Ohio State University's Yu Su presented a concept introducing LLMs as agents that can learn to utilize external tools effectively, effectively expanding their functional toolkit through trial and error simulations.
Advancements in Multilingual Models
The workshop also highlighted the importance of multilingual understanding. Researchers explored strategies to adapt English-pretrained models for low-resource languages. Yen Yu from Apple demonstrated a novel approach using minimal fine-tuning to adapt LLMs for languages with scarce data, reflecting a step toward inclusivity in LLM applications.
Naoaki Okazaki from the Tokyo Institute of Technology presented similar findings for Japanese, elucidating techniques to augment model vocabularies and improve processing speeds without compromising existing capabilities.
Alignment and Security Challenges
As LLMs become integrated into everyday applications, ensuring their alignment with human values and minimizing biases is increasingly critical. Hadas Kotek and Hadas Orgad revealed alarming data on gender biases present in existing models, stressing the necessity for improved classifier probes to detect and mitigate such biases.
Moreover, David Q. Sun talked about a new dataset targeting controversial questions, which aims to evaluate and enhance LLMs' handling of complex, sensitive issues. Techniques for managing outputs related to hallucinations and lack of factuality were also part of the discussion, led by Tatsunori Hashimoto.
On the security front, challenges like jailbreaks and prompt injections were addressed by Chaowei Xiao, who outlined various defense strategies to safeguard against these vulnerabilities.
Conclusion: The Future of NLU is Here
Apple’s workshop on Natural Language Understanding not only showcased the incredible advancements in LLMs but also underlined the collaborative efforts required to ensure their safe and effective application in real-world scenarios. As technology continues to evolve, the emphasis on user interaction and ethical considerations will play a pivotal role in shaping the landscape of artificial intelligence.
Stay tuned as these groundbreaking discussions pave the way for the next generation of intelligent applications—your devices are about to get smarter!