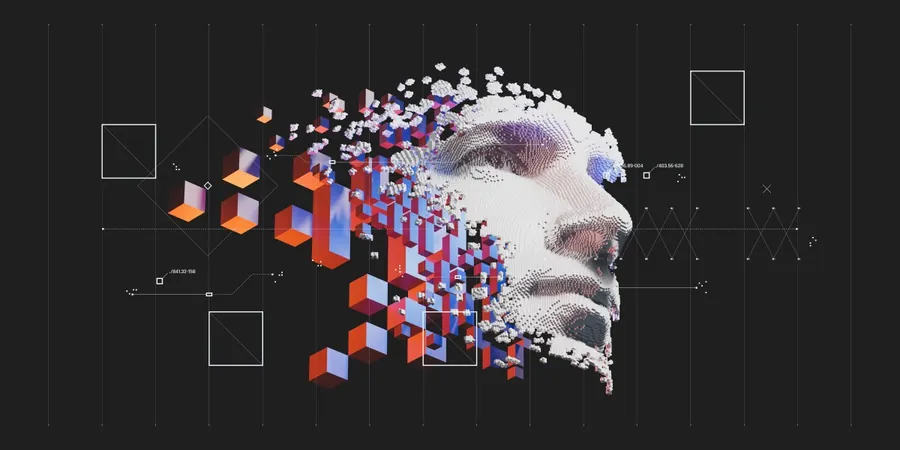
Unveiling the Double-Edged Sword of Synthetic Data in AI Training
2024-12-24
Author: Ming
Is it really possible for AI to learn solely from data generated by another AI? While this might seem like a far-fetched notion, it’s a concept that has gained ground, particularly as the acquisition of new, authentic data becomes more challenging. The use of synthetic data is increasingly becoming a mainstream practice in AI development.
Companies like Anthropic, Meta, and OpenAI are leading the charge by incorporating synthetic data into the training of their high-performance AI models. For instance, Anthropic used synthetic data to enhance one of its models, Claude 3.5 Sonnet, while Meta fine-tuned its Llama 3.1 models with AI-generated data. OpenAI is reportedly leveraging synthetic datasets from its reasoning model, o1, for its upcoming Orion. But why is human-generated data facing a decline, and can synthetic data truly fill this need?
The Critical Role of Data Annotation
AI systems primarily operate as statistical machines, learning from an abundance of labeled examples to discern patterns and make predictions. Annotations—text labels that define the meaning and components of data—are crucial when training these models.
Take, for example, a model that identifies kitchen images. If shown numerous photos labeled "kitchen," it learns to associate that term with typical kitchen features like stoves and sinks. However, labeling inaccuracies can lead to erroneous learning; supplying the label "cow" would prompt the model to misidentify kitchens as cows, highlighting the fundamental necessity for precise annotations.
The demand for annotated data has skyrocketed, leading firms to rely on specialized annotation companies. According to Dimension Market Research, this market is currently valued at $838.2 million and is projected to surge to $10.34 billion within a decade. Yet, while some annotation jobs may pay well—especially those requiring specialized skills—many workers, particularly in developing countries, earn meager wages without job security.
The Conundrum of Data Scarcity
Real-world data sourcing is becoming increasingly arduous. The current climate has made many data owners reluctant to share their resources due to fears of copyright infringement or lack of proper acknowledgement. An alarming trend is that approximately 35% of the top 1,000 websites now hinder OpenAI's data scraping efforts, and a recent study found that around 25% of high-quality data is inaccessible for training models.
If this trend persists, Epoch AI warns that developers could exhaust their data sources for training generative AI models between 2026 and 2032. Coupled with rising legal concerns and the risks of including inappropriate content in datasets, the reality is pushing AI companies to explore synthetic data as a substantial alternative.
The Promising Potential of Synthetic Data
Synthetic data seems to present a perfect solution to these challenges. It offers the prospect of generating diverse and abundant labeled datasets. As Os Keyes, a researcher at the University of Washington, pointed out, synthetic data acts like "biofuel" in the data economy—easily produced without the detrimental effects associated with real-world data.
Recent advancements reflect this trend in the AI industry. Writer, a generative AI enterprise, introduced its Palmyra X 004 model, which is predominantly trained on synthetic data at a relatively low cost compared to traditional methods. Other major players like Microsoft and Google are also utilizing synthetic data in their model developments.
Currently, the market for synthetic data is thriving, with predictions estimating it could grow to a value of $2.34 billion by 2030. Notably, researchers like Luca Soldaini from the Allen Institute for AI reveal that synthetic techniques can create training imprints in formats not easily accessible via conventional data scraping.
The Hidden Dangers of Reliance on Synthetic Data
However, synthetic data isn’t without its pitfalls. Issues such as "garbage in, garbage out" can seriously undermine the quality of AI performance. If the training data for generative models is skewed or biased, the synthetic data they produce will share these flaws, potentially leading to models that reflect inadequate or unrepresented demographics.
Furthermore, studies have highlighted that simply relying on synthetic data might compromise the quality and diversity of AI models in the long run. Researchers from Rice University and Stanford found that overdependence on synthetic datasets during training can lead to deteriorating effectiveness, with a notable decline in variability over generations.
Moreover, complex AI models may produce intricate hallucinations in their synthetic outputs, creating additional layers of ambiguity and reducing the operational accuracy for AI applications. A cascading effect of adverse data from flawed synthesizations could further feed into generational decay of model efficacy.
A Call for Caution and Hybrid Approaches
While some assert that synthetic data may someday achieve self-sustaining capabilities for AI training, today, the technology remains insufficient. OpenAI's CEO Sam Altman has suggested that AI might eventually produce synthetic data robust enough to enable self-training, but no lab has yet released a model trained purely on synthetic data.
For now, relying on human annotators for oversight and curation is essential to ensure the integrity of AI models. The path ahead demands a careful blend of synthetic data with real-world datasets, alongside rigorous quality control measures to avert a potential downward spiral in artificial intelligence capabilities.
The evolution of AI is thrilling and full of potential, but as the landscape shifts, maintaining a balanced approach to data utilization will be vital in harnessing its true power. Synthetic data can be a powerful ally but may also lead to unforeseen consequences if not handled with the utmost care.