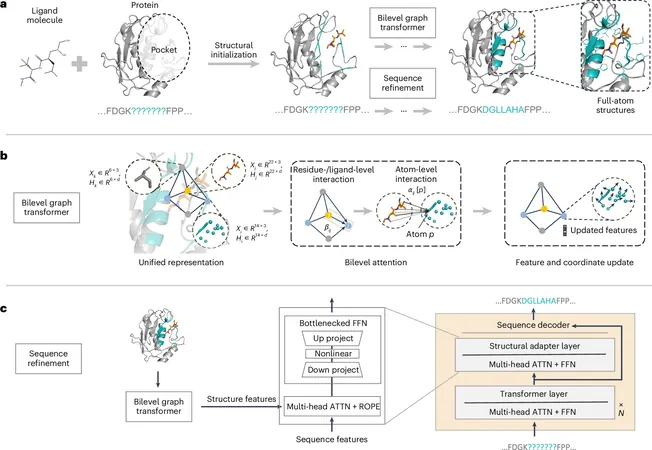
Breakthrough Algorithm Revolutionizes Functional Protein Design, Surpassing Traditional Techniques!
2024-12-09
Author: Amelia
Introduction
In a groundbreaking development, researchers from the University of Science and Technology of China (USTC) and Harvard Medical School have introduced a new deep generative algorithm known as PocketGen. This innovative tool, developed under the leadership of Professor Liu Qi and in collaboration with Marinka Zitnik's lab, has demonstrated remarkable efficiency in generating protein pocket sequences and spatial structures that facilitate binding with small molecules. The study detailing this advancement was published in the highly respected journal, *Nature Machine Intelligence*.
Importance of Functional Protein Design
The significance of functional protein design cannot be understated, especially in the realms of drug discovery and biomedical applications. Proteins that bind effectively to small molecules, such as enzymes and biosensors, are essential for developing new therapeutics. Traditional methodologies, focused on energy optimization and template matching, are often laborious and yield disappointing success rates, leaving a gap in efficient protein design.
Challenges with Existing Techniques
While deep learning approaches have been applied to this field, they encounter difficulties in accurately modeling complex interactions between molecules and proteins, as well as capturing the dependencies between sequence and structure. PocketGen rises to these challenges by providing a solution that boasts both high efficiency and accuracy while remaining true to physicochemical principles.
Algorithm Components
The algorithm builds upon earlier models like FAIR and PocketFlow, incorporating two essential components. First is a dual-layer graph Transformer encoder that draws inspiration from the hierarchical structures of proteins. This feature diligently learns nuanced interaction details, updating both the representations and spatial arrangements of amino acids and atoms to reflect accurate molecular dynamics.
The second component is an advanced pre-trained protein language model. PocketGen adeptly fine-tunes the ESM2 model, enhancing its ability to predict amino acid sequences. By utilizing selective parameter adaptations, it fosters improved sequence-structure consistency through sophisticated cross-attention mechanisms.
Experimental Validation
Experimental validation has shown that PocketGen far surpasses traditional techniques in various aspects, including binding affinity, structural plausibility, and computational efficiency. In particular, it achieves a staggering tenfold increase in processing speed. The algorithm’s effectiveness was particularly evident in tasks involving the design of protein pockets for small molecules such as fentanyl and ibuprofen, outperforming leading generative models like RFDiffusion and RFDiffusionAA, initiatives spearheaded by Nobel Laureate David Baker.
Interpretability and Future Implications
Moreover, PocketGen's attention matrices were rigorously compared with results from established first-principle-based force field simulations, revealing that this deep learning approach not only excels in performance but also offers impressive interpretability, an essential aspect for researchers looking to understand molecular mechanisms.
As the need for efficient and innovative protein design grows amidst the rising challenges of drug resistance and the demand for personalized medicine, advancements like PocketGen could pave the way for a new era in therapeutic development. The implications of this research could be transformational, opening doors to rapid drug discovery processes that save time and resources while delivering more accurate results. Stay tuned—this is just the beginning of a protein design revolution!