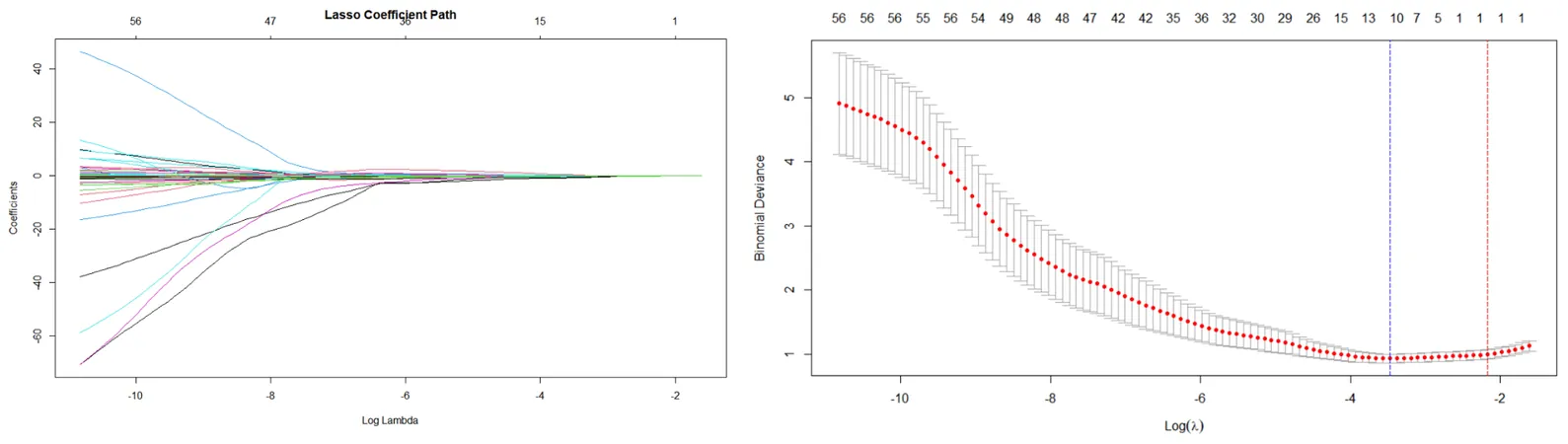
Revolutionary Machine Learning Model Predicts Functional Cure in Chronic Hepatitis B Patients Treated with Pegylated Interferon Alpha
2024-12-23
Author: Emma
Introduction
Chronic Hepatitis B virus (HBV) infection is a pressing global health concern, affecting approximately 296 million people worldwide, with an alarming annual death toll of around 820,000 due to severe liver diseases, including cirrhosis and hepatocellular carcinoma. The HBV primarily targets the liver, evading the immune system by converting relaxed circular DNA into covalently closed circular DNA (cccDNA), a crucial step that contributes to the persistence of the infection.
Treatment Goals and Challenges
For HBV treatment, the ultimate goal is to eliminate hepatitis B surface antigen (HBsAg), a milestone referred to as a "functional cure." Currently, the main antiviral therapies include nucleos(t)ide analogs (NAs) and pegylated interferon alpha (PEG-INFα). While NAs are effective in reducing viral replication, they do not adequately address cccDNA or potently restore the host's immune response. This shortcoming often leads to rebounds in viral activity post-treatment, causing significant liver damage.
In contrast, PEG-INFα therapy has shown more promise, often leading to greater reductions in HBsAg levels and potentially higher rates of clearance even after treatment ceases. However, the benefits of PEG-INFα are tempered by limitations such as adverse side effects, high costs, and the necessity for regular subcutaneous injections. Only a select group of patients can attain a functional cure with this treatment, underscoring the importance of early predictors to assess treatment responsiveness.
Predictive Factors and the Need for a Model
Recent research indicates that various clinical factors—such as fluctuations in alanine aminotransferase (ALT), HBsAg levels, and intrahepatic cccDNA—can serve as predictors for achieving a functional cure. However, most previous studies have focused on individual parameters, which restricts their predictive capacity. Moreover, many tests involved are either too complex or costly for regular clinical settings, prompting a need for a more streamlined approach.
Machine Learning Approach
This study breaks new ground by developing a machine learning model that analyzes clinical laboratory indicators to forecast HBsAg clearance in chronic hepatitis B patients. Utilizing an extensive dataset from 224 patients treated with PEG-INFα at Mengchao Hepatobiliary Hospital, researchers employed machine learning to uncover patterns across diverse demographic and clinical parameters in order to enhance the accuracy of predictions.
Predictive Model Insights
The predictive model created can estimate the likelihood of achieving a functional cure as early as 12 weeks into treatment, taking into consideration factors such as baseline HBsAg levels, ALT fluctuations, and other immunological markers. The analysis resulted in a performance validation showing a commendable ability to predict treatment outcomes, with an area under the curve (AUC) of 0.862 in the training set and 0.858 in the validation set.
Among the patients followed over 48 weeks, an impressive clearance rate of 25.45% was observed, swaying the odds considerably in favor of those with lower HBsAg levels. The research further demonstrated that as HBsAg levels declined, the chances of achieving a functional cure increased markedly, especially for individuals with levels dipping below 100 IU/ml, where the clearance rate soared to nearly 63.89%.
The model's robustness also lies in the ease of accessing the input variables, as they are common measurements in clinical practice. By identifying patients most likely to benefit from PEG-INFα treatment, healthcare providers can personalize therapy, optimize resource allocation, and potentially improve long-term patient outcomes.
Limitations and Future Directions
Despite these encouraging findings, the study bears some limitations, including its retrospective design and reliance on a single-center patient cohort, which could affect the generalizability of the results. Future research should seek to validate the model's predictive capabilities across larger, multicenter studies and further investigate genetic factors and other predictors of treatment efficacy.
This advancement in leveraging machine learning to assess treatment responses opens new pathways for managing chronic hepatitis B, aiming not only to enhance patient care but also to alleviate the broader public health burden posed by this insidious virus. As we continue to refine these predictive tools, the potential to revolutionize hepatitis B management becomes increasingly tangible.