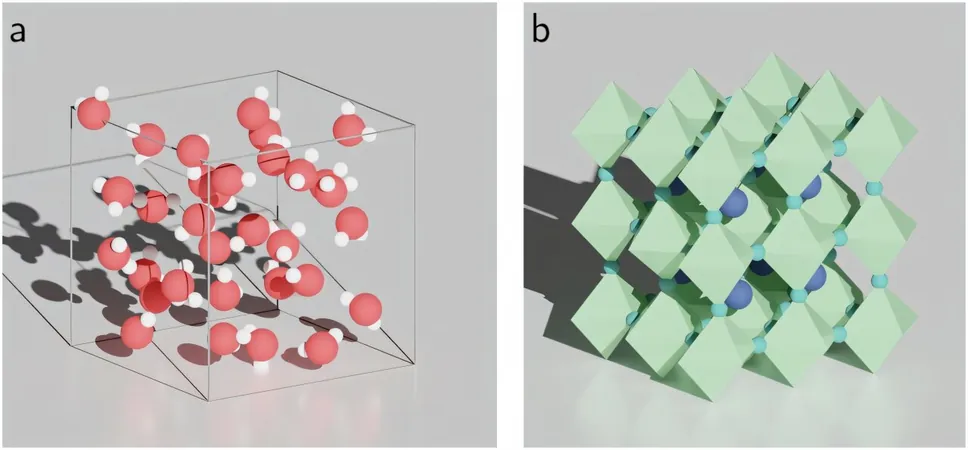
Revolutionizing Materials Science: How Machine Learning Is Shattering Boundaries in Spectral Property Predictions!
2024-12-23
Author: Olivia
In the realm of computational materials science, unraveling the mysteries of materials' spectral properties has usually been an arduous task, often requiring scientists to sift through extensive calculations to identify parameters that encapsulate the unique physics of different materials. This traditional process demands enormous amounts of time and computational resources, prompting researchers to seek faster and more efficient alternatives.
Among the innovative approaches emerging in this field are Koopmans functionals. This promising technique enhances density-functional theory (DFT), enabling predictions not only of ground state properties—like atomic positions—but also the spectral properties, which dictate how materials interact with light. From knowing what frequencies a material absorbs to how it behaves in devices like solar cells, understanding these properties is crucial for advancements in technology.
“The screening parameters are pivotal,” says Edward Linscott, a postdoc at the Paul Scherrer Institute and a key contributor to this research. He explains that these parameters measure the extent to which electrons in a system adjust when an additional electron is added or removed, a phenomenon essential for analyzing the spectral responses in materials.
The term "screening" aptly describes this process, where other electrons obscure the view of an added or removed electron, complicating the analysis. Linscott clarifies, "In phenomena like solar cells, shining light on photovoltaic material ejects electrons, generating electrical currents. However, DFT struggles to accurately describe these events, necessitating understanding the strength and dynamics of electronic screening.”
While Koopmans functionals offer depth in analysis, the downside has been their time-intensive calculations, particularly in estimating the screening parameters. Fortunately, a groundbreaking study published in *npj Computational Materials* illustrates that a straightforward machine learning model can drastically streamline these calculations. Led by Yannick Schubert from the University of Zurich and his research team, this work aims to harness machine learning to reduce the time spent on calculating screening parameters within the Koopmans framework.
The researchers focused on two intriguing materials: liquid water and the halide perovskite CsSnI3. Liquid water represents an intricate system marked by disorder, while CsSnI3 shines as a frontrunning candidate for solar cells, where understanding temperature fluctuations is vital.
To effectively model the spectral properties using Koopmans functionals, earlier methods would demand numerous calculations on variations of the same chemical system. By training a machine learning model on a selective subset, the researchers have found a means to extrapolate screening parameters for the remaining configurations more rapidly.
The initial challenge was determining which machine learning model would yield optimal results for their needs. Surprisingly, after considering more complex alternatives, they discovered that a simple ridge regression model performed exceedingly well even with limited data.
“We were prepared to experiment with advanced networks,” Linscott notes. “Yet, we were astonished that such a straightforward model sufficed and delivered reliable screening parameters.”
The triumph of their methodology is not solely attributed to the model but also to the researchers' meticulous design of "descriptors"—mathematical constructs that encapsulate the relevant physics of the system at hand, which are then fed into the machine learning algorithm.
Looking ahead, there exists potential to enhance this approach with more sophisticated networks and larger datasets. However, Linscott suggests that the immediate focus should be on maximizing the insights gained from the current method to probe temperature-dependent spectral properties of compelling materials.
As it stands, this innovative leap could pave the way for rapid advancements in materials science, particularly in developing next-generation technologies, such as more efficient solar cells and other optoelectronic devices. The fusion of machine learning with materials science is poised to transform our understanding of materials and accelerate discoveries in the field—are we on the verge of a new scientific revolution? Stay tuned!