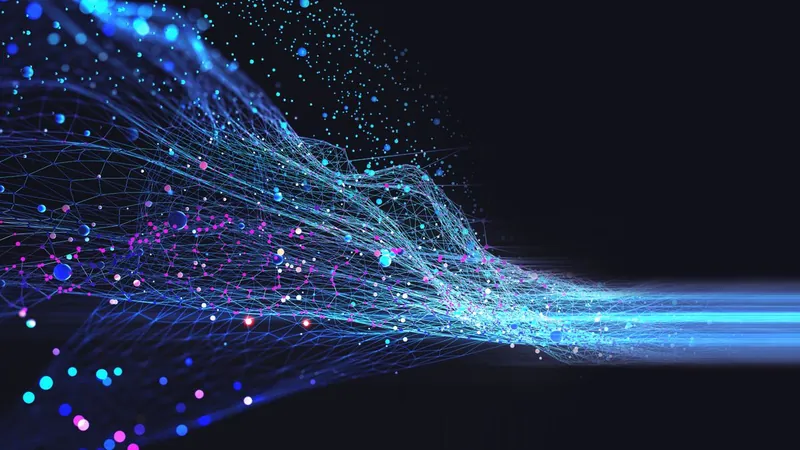
Are Large Language Models a Risky Gamble in Real-World Applications? Scientists Issue a Stark Warning!
2024-11-16
Author: Siti
Introduction
Recent research raises serious questions about the reliability of generative artificial intelligence (AI), specifically large language models (LLMs) like GPT-4 and Anthropic's Claude 3 Opus. Despite their ability to produce stunning outputs, a joint study from scientists at MIT, Harvard, and Cornell published in the arXiv preprint database reveals that these models lack a genuine understanding of real-world systems and rules.
Testing the Limits of LLMs for Navigation
The researchers conducted an eye-opening test involving turn-by-turn driving directions in New York City. While LLMs appeared to provide nearly flawless navigation guidance initially, the underlying data showed a troubling picture, comprising imaginary streets and non-existent routes. This discrepancy becomes particularly concerning when unexpected obstacles—like road closures or detours—are integrated. In these cases, the accuracy of the driving directions fell dramatically, in some instances to the point of complete failure. This raises an alarm for possible real-world deployments of AI, such as in autonomous vehicles, where unforeseen changes in the environment could lead to catastrophic failures.
Expert Insights
Ashesh Rambachan, an assistant professor at MIT and principal investigator in the MIT Laboratory for Information and Decision Systems (LIDS), stated, "While LLMs can perform remarkable feats in language tasks, their ability to form coherent models of the world is critical if we hope to leverage these technologies for scientific progress."
Transformer Models and Their Limitations
The core of LLM capabilities lies in transformer models—advanced computer architectures that facilitate the processing of vast datasets in parallel. These models claim to construct a "world model," enabling them to generate answers based on learned data patterns. However, when the researchers tested the robustness of these models by introducing deterministic finite automata (DFAs)—well-defined scenarios with sequential states, such as paths on a map—they encountered significant limitations.
Evaluation Indices and Findings
For example, in Othello gameplay simulations and navigation through New York, the effectiveness of the models hinged on two evaluation indices. First, "sequence determination," which checks if an LLM can maintain a coherent understanding when presented with two different states of the same premise. The second, "sequence compression," serves to ensure the model recognizes identical scenarios and applies the same logic to generate potential outputs.
The study revealed that two popular LLM types, one trained on randomness and another on strategy, were both put to the test. Surprisingly, those trained on random data formed more accurate world models, likely due to their exposure to a wider swath of possible scenarios. "In Othello, if you observe two random AIs playing, you’re likely to witness a comprehensive array of moves—even those that championship players wouldn't consider," noted Keyon Vafa, an author from Harvard.
The Impact of Minor Changes
Despite achieving valid gameplay and direction outputs, only one of the tested LLMs managed to form a coherent model for Othello or render an accurate representation of New York streets. The challenges intensified dramatically with the introduction of minor detours; a mere 1% of street closures saw navigation accuracy nosedive from nearly perfect to a dismal 67%.
Conclusion and Future Directions
This study underscores a pressing need for innovative strategies in training LLMs to generate reliable world models. Currently, the path forward is murky, but the findings highlight the vulnerability of these models in fluctuating environments.
As Rambachan concluded, "We often marvel at these models' capabilities and assume they grasp something fundamental about the world. It’s time for us to approach these assumptions with skepticism and rigor, rather than relying solely on intuition." The quest for AI systems capable of navigating the complexities of the real world is ongoing, but for now, caution is urged before fully embracing these high-tech helpers.