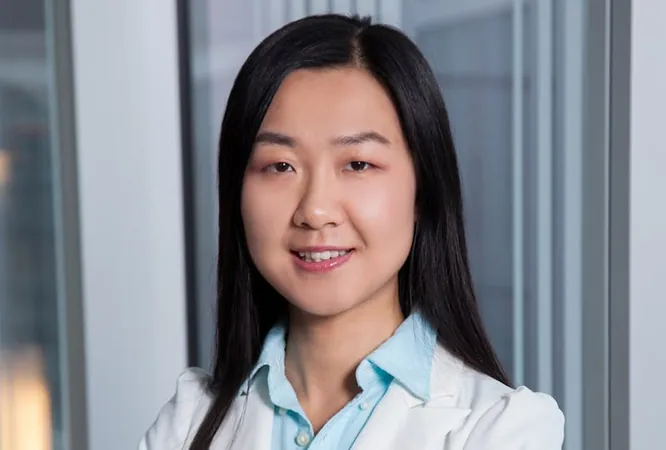
Game-Changer in Autonomous Vehicle Safety: Multi-Agent Learning Takes the Stage!
2024-11-17
Author: Jia
Introduction to Multi-Agent Reinforcement Learning (MARL)
In a groundbreaking presentation on November 8, Fei Miao, an Associate Professor at the University of Connecticut's School of Computing, introduced innovative advances in Multi-Agent Reinforcement Learning (MARL) targeted at Connected and Automated Vehicles (CAVs). Her talk, entitled “Learning and Control for Safety, Efficiency, and Resiliency of Embodied AI,” revealed how her research aims to revolutionize autonomous driving by improving communication, decision-making, and safety among multiple vehicles.
What is Embodied AI?
So what exactly is Embodied AI? It’s an advanced form of artificial intelligence that learns and interacts with its surroundings, especially when multiple autonomous vehicles need to coordinate—think of a symphony where every musician has to be in tune! Miao emphasized the importance of perfect coordination among these vehicles, particularly in dynamic environments such as busy roadways, manufacturing facilities, and drone operations.
Is Your Safety at Risk? The Real-World Challenge of Autonomous Vehicles!
One critical concern in MARL is safety. While simulating training environments for reinforcement learning often allows for simple restarts after failures, the stakes are astronomically higher for real-world autonomous vehicles. A miscalibration in these systems could pose a serious danger to human lives. Miao starkly pointed out the risks involved: “If an autonomous vehicle fails in real life, then it will be dangerous for human life.”