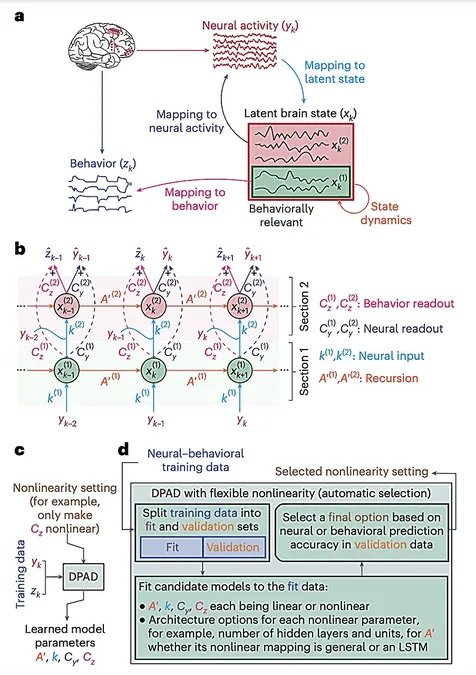
Groundbreaking RNN Framework Revolutionizes Our Understanding of Neural Dynamics and Behavior
2024-10-05
Groundbreaking RNN Framework Revolutionizes Our Understanding of Neural Dynamics and Behavior
In a pivotal stride for neuroscience, researchers from the University of Southern California and the University of Pennsylvania have unveiled a cutting-edge framework utilizing Recurrent Neural Networks (RNNs) to model the complex dynamics of neuron populations, shedding light on how these dynamics influence human and animal behaviors. Traditional approaches relying on static images and scans have often provided an incomplete picture, failing to capture the intricate changes in neural activity over time.
Historical Models and Limitations
Historical models predominantly employed linear dynamics, which assume that alterations in neural activity follow straightforward patterns. While these models are simpler to interpret, they frequently fall short when it comes to depicting the multifaceted nature of actual neural dynamics. This limitation has spurred scientists to explore more intricate, nonlinear models capable of accounting for diverse neuronal behaviors.
The DPAD Framework
The breakthrough framework, known as Dissociative Prioritized Analysis of Dynamics (DPAD), allows researchers to differentiate between behaviorally relevant neural dynamics and general neural activity. This is an essential development, as understanding how brain functions translate into behavior is increasingly recognized as crucial in neuroscience research.
Publication and Validation of DPAD
In their recent publication in Nature Neuroscience, authors Omid G. Sani, Bijan Pesaran, and Maryam M. Shanechi detailed the advantages provided by their RNN-based framework, which is adept at isolating and prioritizing behaviorally relevant latent states while processing expansive datasets of neural activity. Utilizing a robust four-step optimization algorithm, DPAD can enhance the predictive accuracy of neural behaviors significantly.
Research Applications
To validate their model’s capabilities, the researchers applied it to five distinct problems in neuroscience, focusing on the neural activity of non-human primates as they engaged in various tasks. They reported that DPAD outperformed traditional models, particularly in its ability to identify nonlinear transformations related to behavioral predictions. Not only did DPAD demonstrate more accurate neural-to-behavior predictions, but it also facilitated nonlinear dimensionality reduction, thereby allowing a deeper exploration of how latent cortical dynamics inform behavior.
Implications and Future Research
This innovative approach provides a comprehensive method for analyzing the dynamics of neural behavior interactions. Their findings indicate that significant nonlinearities in neural dynamics primarily influence the transformation of latent dynamics into observable behaviors, offering fertile ground for further investigation.
Broader Applications of DPAD
The implications of this research extend beyond primate studies. The versatility of the DPAD framework suggests that it could be employed to explore neural dynamics across various scenarios, including the activity different brain regions exhibit in response to sensory stimuli and during various cognitive tasks. This flexibility establishes DPAD as a groundbreaking tool in the toolkit of neuroscientists seeking to unlock the intricate dance between neuronal dynamics and behavior.
Conclusion
As the scientific community begins to embrace this novel modeling framework, it holds promise for revolutionizing our understanding of the brain's operations, opening doors to new hypothesis testing, and potentially leading to advancements in treating neurological disorders linked to aberrant neural dynamics.
Keep an eye on this exciting development in neuroscience - who knows what other secrets the brain may hold!