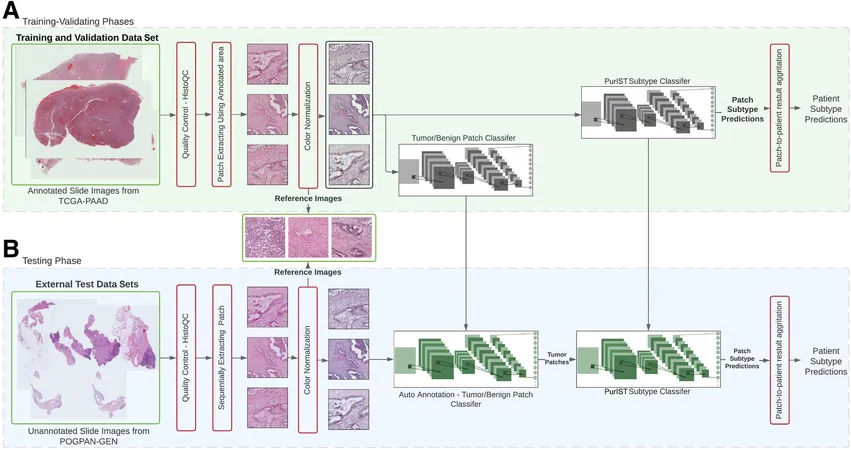
Revolutionary AI Tool Transforms Pancreatic Cancer Diagnostics: A Game Changer for Early Detection!
2024-12-12
Author: Rajesh
Introduction
In a groundbreaking study published in the American Journal of Pathology, researchers have introduced an innovative deep learning model designed to classify pancreatic ductal adenocarcinoma (PDAC), the most prevalent form of pancreatic cancer, into distinct molecular subtypes utilizing histopathology images. This cutting-edge technique not only boasts remarkable accuracy but also serves as a rapid and cost-efficient alternative to conventional diagnostic methods that typically rely on expensive molecular assays.
The Need for Early Detection
The urgency of this advancement is underscored by alarming statistics: PDAC has now overtaken breast cancer as the third leading cause of cancer-related deaths in Canada and the United States. While surgical intervention can effectively cure approximately 20% of PDAC patients if diagnosed early, the overarching five-year survival rate lingers at a mere 20%. Tragically, around 80% of individuals are diagnosed with metastatic disease, often succumbing to the illness within just a year.
Challenges in Patient Care
The inherent aggressiveness of PDAC presents significant challenges when it comes to determining effective patient care plans via sequencing technologies. The rapid deterioration associated with this cancer necessitates immediate identification of eligible patients for targeted therapies and clinical trials. However, the current turnaround times for molecular profiling—stretching from 19 to 52 days post-biopsy—leave much to be desired in meeting the urgent clinical needs of these patients.
Expert Insights
Dr. David Schaeffer, co-lead investigator from the University of British Columbia's Department of Pathology and Laboratory Medicine, remarked, 'With the discovery of more potentially actionable subtypes for personalizing treatment in pancreatic cancer, we face the challenge of our methods, which predominantly rely on genomic analysis of extracted DNA and RNA from tissue samples. This approach can be exceptionally effective but often falls short due to the limited availability of tissue from PDAC tumors, which are notoriously difficult to sample.'
AI-Powered Diagnostic Methodology
The researchers' new AI model utilizes routine hematoxylin and eosin (H&E) stained slides, a widely available and cost-effective diagnostic technique routinely performed in pathology labs, thereby significantly enhancing clinical management of PDAC. By employing deep learning AI, the team trained models on whole-slide pathology images to differentiate between two molecular subtypes: basal-like and classical PDAC.
Model Performance
Through extensive testing, the best-performing model showcased a stellar accuracy rate of 96.19% in identifying these subtypes using data from The Cancer Genome Atlas (TCGA) and an accuracy of 83.03% within a local cohort. This demonstrates the model’s robustness across varied datasets.
Impact on Patient Triaging
Dr. Ali Bashashati, another co-lead investigator at UC's School of Biomedical Engineering, emphasized the implications of their findings: 'Our model exhibited outstanding sensitivity and specificity rates of 85% and 100%, respectively, making it a highly valuable tool for triaging patients for molecular testing. Notably, the AI's capability to accurately identify subtypes directly from biopsy images presents a pivotal advance, facilitating immediate applications at the time of diagnosis.'
Conclusion
As we move forward into an era where AI-driven advancements hold the potential to reshape cancer diagnostics and patient management, this revolutionary tool stands as a beacon of hope for improving outcomes in the battle against pancreatic cancer. The continued exploration of these technologies may pave the way for a future where swift, precise treatments become the new standard, potentially saving countless lives. Stay tuned for further developments in this exciting field!