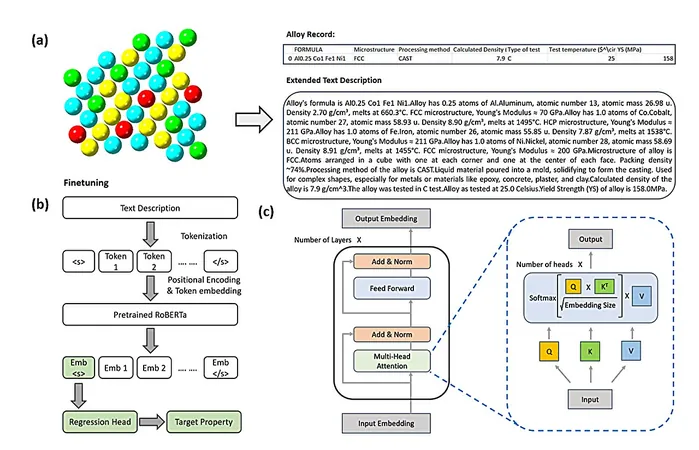
Revolutionary AlloyBert Model Set to Transform Alloy Property Identification
2025-01-10
Author: Arjun
Introduction
Identifying the properties of metal alloys can often feel like both an art and a science, blending complexity and costs unpredictably. Traditional methods for determining these properties, such as conducting experiments or using Density Functional Theory (DFT) calculations, are notorious for being both time-consuming and resource-intensive. Enter AlloyBert—a groundbreaking transformer-based model developed by Amir Barati Farimani and his dedicated research team, designed to streamline this intricate process.
How AlloyBert Works
AlloyBert works by taking simple English descriptors as inputs, such as the chemical composition and processing temperatures of alloys. By removing the necessity for highly precise wording, it provides flexibility—a crucial attribute that could revolutionize the field of materials science. According to Akshat Chaudhari, a master's student in materials science and engineering and part of the AlloyBert team, "We aimed to create a model that can detect specific physical properties without the users needing to stress over exact phrasing."
Technical Details
The model is built upon RoBERTa, a sophisticated pre-existing encoder that utilizes a self-attention mechanism, enabling it to determine the importance of individual words in a sentence. This innovative structure allows AlloyBert to predict key properties of alloys, including yield strength and elastic modulus.
Performance Observations
However, despite its potential, the research team observed a couple of intriguing deviations in AlloyBert's performance. Surprisingly, results have shown that sometimes the least detailed input can yield the most accurate predictions. This unexpected finding raises questions about the training datasets, which currently encompass only two sets of alloy properties. Chaudhari points out, "Training the model on a more extensive dataset could lead to more reliable results."
Training Strategies
Another area of exploration involves the model's training strategies. The researchers utilized two methods: one that involved a two-step process of pre-training followed by fine-tuning, and another that focused solely on fine-tuning. Although the two-step method showed potential, the results varied significantly, with the latter approach achieving superior accuracy in some cases. This inconsistency may stem from how the pre-training was initially conducted, utilizing a Masked Language Model (MLM).
Future Applications
In addition to addressing the existing deviations, the potential applications for AlloyBert are boundless. The development team envisions enhancements that would extend beyond alloys to include a wider variety of materials. There's also ambition to create a companion model capable of reverse operations: given an alloy property, it would deduce the elements that make up the alloy.
Implications for the Future
The implications of AlloyBert and similar transformer-based models are far-reaching. As Chaudhari notes, "In scientific research, having accurate and concrete answers is paramount. Current research shows a significant scope for improvement, and models like AlloyBert can be optimized to surpass traditional methodologies."
Conclusion
As the fields of material science and engineering increasingly embrace artificial intelligence, AlloyBert is poised to become an indispensable tool for researchers worldwide. With ongoing advancements, the quest for efficient alloy property identification is reaching new horizons, promising to elevate scientific inquiry and industrial applications to unprecedented levels.