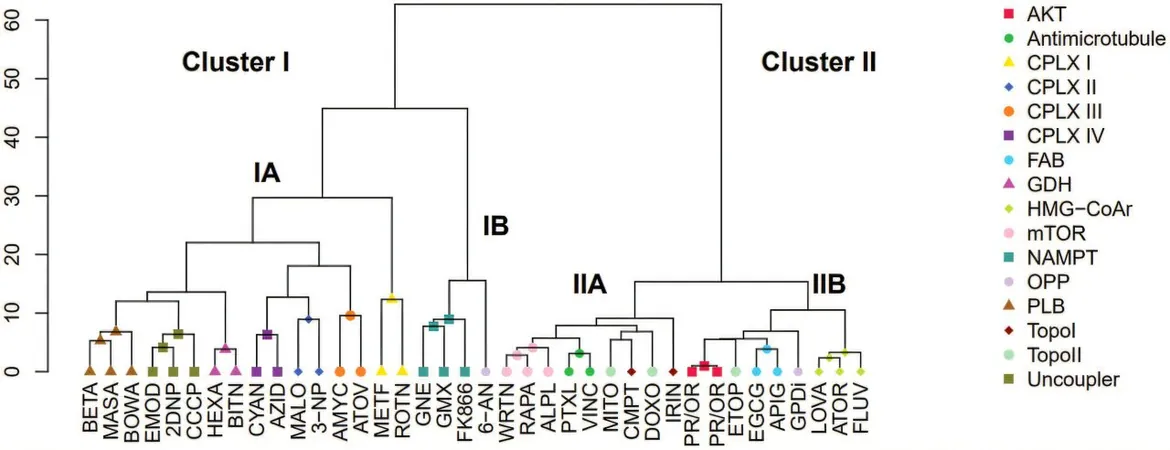
Revolutionizing Anti-Cancer Drug Development: AI Predicts Mechanisms of Action
2024-11-19
Author: Daniel
Introduction
Recent advances in the field of cancer research are shedding light on a groundbreaking approach that leverages artificial intelligence (AI) to predict the mechanisms through which new anti-cancer compounds operate. Researchers from the Leibniz Institute of Plant Biochemistry, in collaboration with the Martin Luther University Halle-Wittenberg, have made significant strides in addressing one of the biggest hurdles in the development of effective anti-cancer therapies—understanding how these compounds affect cancer cells.
The Role of Natural Compounds
Plants, fungi, and bacteria are known for producing a wealth of bioactive compounds, many of which have the potential to disrupt cellular processes and could lead to innovative cancer treatments. However, one of the major challenges in developing new anti-cancer drugs is deciphering their modes of action, which has traditionally been a time-consuming process.
Targeting Cancer Metabolism
Cancer cells exhibit unchecked growth and possess a markedly elevated metabolic rate, requiring vast amounts of energy and building blocks for cell division. This aberrant metabolic behavior sets them apart from normal cells and presents an attractive target for therapeutic intervention. Most established anti-cancer drugs exploit this characteristic by influencing the tumor's hyperactive metabolism, ultimately leading to the death of these rapidly dividing cells. This can involve blocking the uptake of glucose, inhibiting enzymes crucial for energy production, or interfering with DNA replication.
Study Findings
In their cutting-edge study, scientists analyzed the metabolite profiles of prostate cancer cells treated with 38 different cytotoxic compounds, including several well-known anti-cancer drugs, to establish a relationship between the drugs and the resulting metabolite patterns. Their hypothesis was straightforward: compounds targeting the same cellular proteins would produce similar metabolite profiles. After rigorous testing, the findings confirmed that tumor inhibitors with analogous mechanisms produced consistent metabolite signatures.
AI Model Development
This powerful correlation informed the training of an AI model, which was subsequently employed to predict the mechanisms of action for new natural plant-derived substances known to have anti-cancer properties. For instance, compounds like 11-keto-β-boswellic acid from frankincense and maslinic acid from olives were identified as capable of disrupting cancer energy metabolism and inducing cell death.
Validation of Predictions
As proof of concept, the AI predictions were validated across different cancer cell models, including breast cancer and Ewing's sarcoma cells, where the AI also demonstrated its predictive capabilities, albeit with slightly reduced accuracy due to a lack of initial training data for those specific cell types.
Implications for Future Research
Beyond simply aiding in the discovery of new anti-cancer drugs, this innovative integration of metabolomics and AI could also refine our understanding of existing chemotherapeutics, revealing previously unknown effects or interactions. Such insights are invaluable, particularly in the context of combination chemotherapy, where multiple agents are used concurrently to enhance treatment efficacy.
Conclusion
As researchers continue to expand their training datasets and explore various cancer types, the hope is that this predictive approach will expedite the identification of effective therapies, offering new hope in the battle against cancer. With these promising developments, the landscape of cancer treatment is poised for a transformative shift—can we be on the verge of unleashing a new era of precision medicine? Stay tuned for more updates!