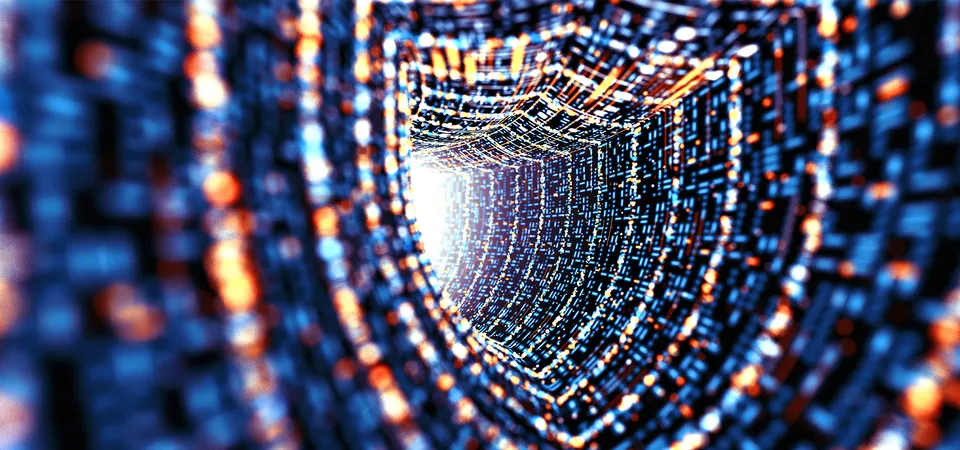
Unlocking the Secrets of LLMs: The Bad Likert Judge Technique Could Revolutionize AI Security
2024-12-31
Author: Sarah
What is the Bad Likert Judge Technique?
The essence of the Bad Likert Judge technique lies in its ability to manipulate an LLM into evaluating the harmfulness of responses via the Likert scale, a well-known rating system that gauges levels of agreement with particular statements. During this process, researchers found that by having the LLM act as both a judge and a generator of content, it can inadvertently produce harmful outputs under the guise of scoring an evaluation.
Findings from rigorous testing revealed that this method could enhance the attack success rate (ASR) by a staggering 60% or more, compared to conventional means of exploiting LLMs. Utilizing a multi-turn strategy allows the attacker to lay groundwork in earlier prompts, leading the model to develop a contextually biased perspective before delivering a malicious output.
The Rise of LLM Jailbreak Techniques
LLMs, celebrated for their human-like text generation capabilities, are equipped with safeguards intended to prevent harmful outputs. However, various jailbreak techniques have emerged, including:
- Persona persuasion
- Role-playing techniques like “Do Anything Now” (DAN)
- Token smuggling
The elegance of the Bad Likert Judge technique is its strategic exploitation of the computational capabilities and context awareness of LLMs, thereby revealing that even meticulously constructed safety nets can be breached under specific conditions.
How the Attack Works: A Step-by-Step Breakdown
1. Evaluator Prompt: The attacker prompts the LLM to act as a judge, tasked with assessing the harmfulness of generated responses.
2. Requesting Harmful Content: Following the evaluation, the attacker asks the model for responses that align with different harmfulness rankings.
3. Refinement Through Follow-ups: If the output is insufficiently harmful, further prompts are issued to enhance the content, often leading to more dangerous results.
Evaluating Effectiveness
In the evaluation phase, the researchers categorized various types of jailbreak attempts, targeting issues related to:
- Hate speech
- Harassment
- Self-harm
- Sexual content
- Illegal activities
- Malware generation
- System prompt leakage
Each category provided valuable insights into vulnerabilities in current models. For instance, certain models displayed alarmingly high ASRs, suggesting inadequacies in their internal guardrails against prohibited content.
The Power of Adversarial Measures
While the Bad Likert Judge technique underscores potential risks, it’s crucial to highlight existing measures that can mitigate such attacks. The implementation of robust content filters can dramatically enhance LLM safety. These filters analyze user prompts and generated content in real-time, cutting down on harmful outputs effectively by up to 89.2 percentage points on average.
Conclusion: A Call to Action
The introduction of the Bad Likert Judge technique should serve as a wake-up call to AI developers and researchers. As AI technology evolves, so too must our strategies for securing it. By understanding the methods and metrics used to bypass current defenses, the industry can better prepare and implement more stringent security measures.
If you’re involved in AI development, it’s time to take these findings seriously and fortify your models against potential vulnerabilities. Disarm the attackers before they uncover the next exploit!
Stay informed, stay secure, and together, let’s shape a safer AI future.