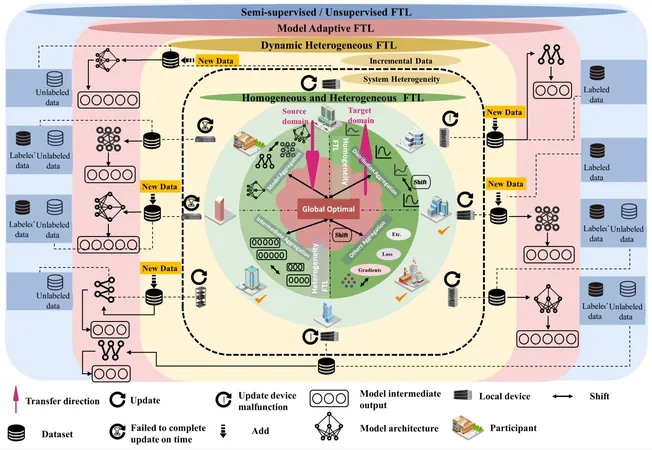
Unveiling the Future of Federated Transfer Learning: Breakthroughs, Challenges, and Practical Applications
2024-12-27
Author: Daniel
Introduction
In an era where data privacy is paramount, Federated Learning (FL) has emerged as a revolutionary paradigm that allows multiple participants to collaboratively train machine learning models without sharing their sensitive local data. However, this innovative approach isn't without its obstacles. Traditional FL techniques struggle with issues like data heterogeneity, variations in system capabilities, and the scarcity of labeled data.
The Emergence of Federated Transfer Learning (FTL)
Enter Federated Transfer Learning (FTL), a groundbreaking integration of Transfer Learning (TL) that is gaining traction among researchers. FTL enhances the FL framework by enabling knowledge sharing across different data sources while safeguarding user privacy. Yet, this dynamic approach also introduces unique complexities that standard TL does not encounter, including the need for continual knowledge sharing during each communication round without compromising local data security.
Recent Research and Insights
To shed light on these developments, a research team spearheaded by Fuzhen Zhuang recently published a pivotal study on December 15, 2024, in the esteemed journal *Frontiers of Computer Science*. This extensive survey categorizes six prevalent scenarios in FTL: homogeneous FTL, heterogeneous FTL, dynamic heterogeneous FTL, model adaptive FTL, semi-supervised FTL, and unsupervised FTL. Through meticulous analysis, the researchers summarize effective strategies for overcoming FTL’s challenges, addressing key factors such as core algorithms, model design, privacy-preserving methods, and communication frameworks.
Real-World Applications of FTL
Beyond theoretical constructs, the study emphasizes real-world applications of FTL across diverse sectors, including healthcare, finance, and smart cities. The authors highlight the crucial role of robust systems and infrastructure in facilitating effective FTL processes and offer insights into current practical implementations that are reshaping industries.
Future Directions and Innovation
Moreover, the researchers propose future directions for innovation and the broader adoption of FTL, aiming to bridge existing gaps while encouraging interdisciplinary collaboration. This comprehensive survey is a treasure trove of knowledge for machine learning practitioners, data privacy advocates, and distributed computing professionals, laying a robust groundwork for the exploration of challenges and opportunities within the realm of FTL.
Conclusion
Stay ahead of the curve in the fast-evolving landscape of artificial intelligence and discover how Federated Transfer Learning could revolutionize data privacy and collaborative model training. Don’t miss out on this chance to be part of the data science revolution!